Unsere vernetzte Welt verstehen
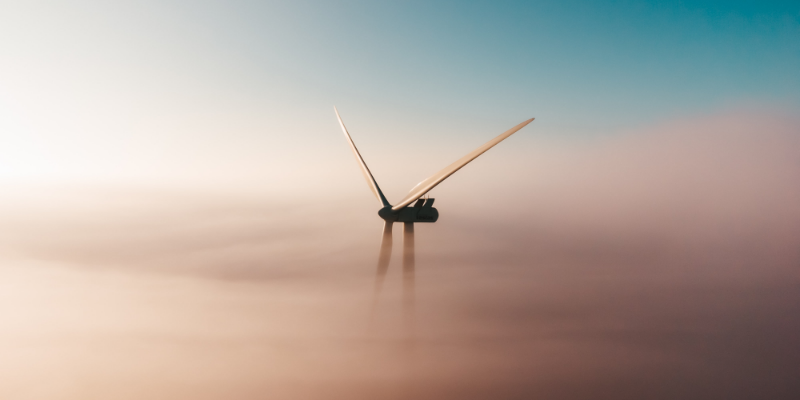
Sustainable AI – Wie nachhaltig ist Künstliche Intelligenz wirklich?
Sustainable AI is becoming increasingly important. But how sustainable are AI models really? Big tech and smaller applications differ greatly in this respect. We have looked at how sustainable small-scale AI really is, what open questions remain and what recommendations can be made.
Sustainable AI and AI for Sustainability
For quite some time now, one can read about the potentials of AI for the fight against climate change. For example, Rolnick et al. (2023) conducted a survey on many (potential) use cases, such as Enabling low-carbon electricity or Managing forests. However, there are also concerns about the sustainability of AI. Often, scientists make a difference between sustainable AI and AI for sustainability. While the latter serves purposes like increasing the efficiency of renewable energy, the former is about making AI itself sustainable; because what often remains unsaid: many AI models consume massive amounts of energy.
The bigger the better
In a widely cited study, Emma Strubell and colleagues conducted experiments with natural language models which found that some of these models emit as much CO2 as five cars in their entire lifetime. In another famous study (Fig 1), researchers at Open AI looked at the growth of AI models in the recent year and found that they double their size every 3.4 months, thus contributing to a striking increase in energy consumption.
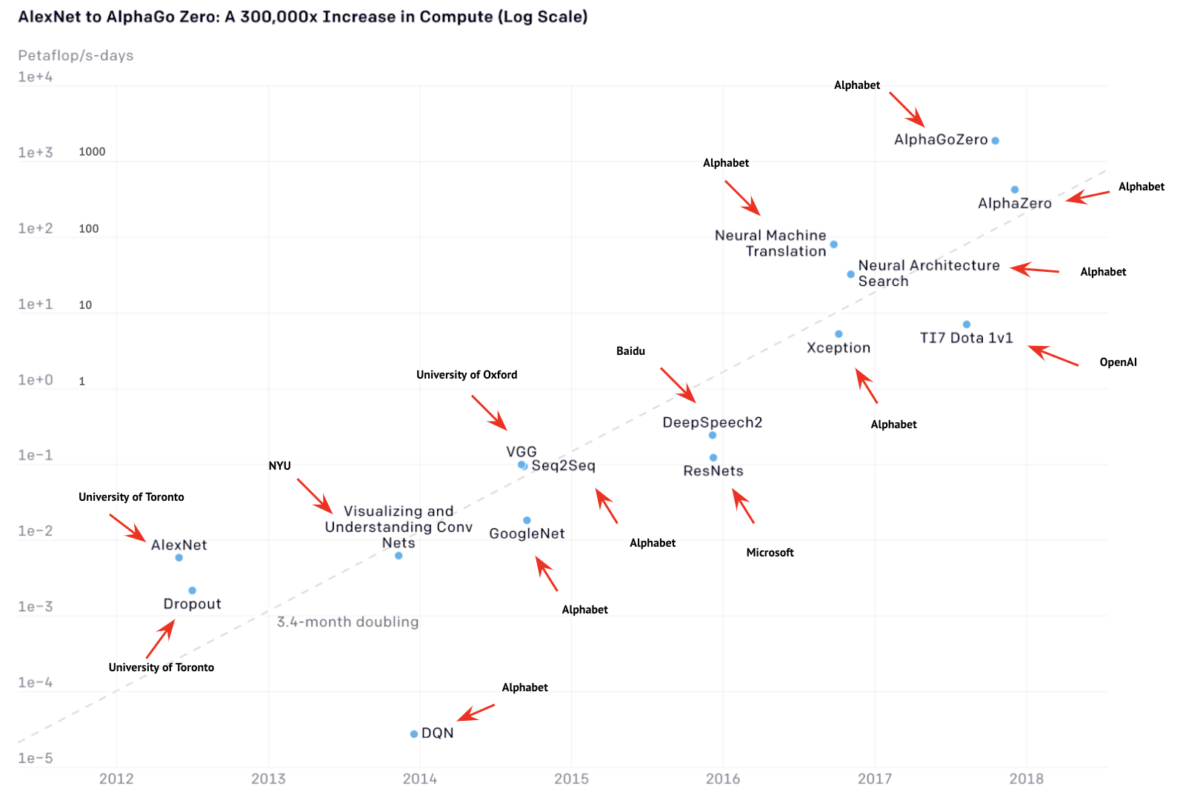
Based on these results, it is often claimed that the current trend to bigger and bigger models is far from sustainable. Without denying this, a closer look at the numbers is startling: It is clear / the data shows that the vast majority of the models in Fig 1 are built by Big Tech companies like Alphabet, Microsoft, or Baidu. As a matter of fact, it is no secret that AI research is not driven by “normal” universities anymore. The reason for this is of financial nature: training such models is extremely costly. For example, Strubell et al (2019) report that the costs for cloud computing can be around $100,000.
Sustainability on Huggingface
Unfortunately, there are no reliable numbers on the energy consumption of smaller AI-projects. The only exception is an experiment conducted by Marcus Voß from Birds on Mars, who did this for the study “Nachhaltigkeitskriterien für künstliche Intelligenz”. We were able to reproduce and update the results (Fig 2). The datasource is the (self-reported) emissions by models found on Huggingface, a hosting platform for AI models. These models can be downloaded and fine-tuned to one’s own purposes, and since the models are freely available, they give some insights into smaller AI projects.
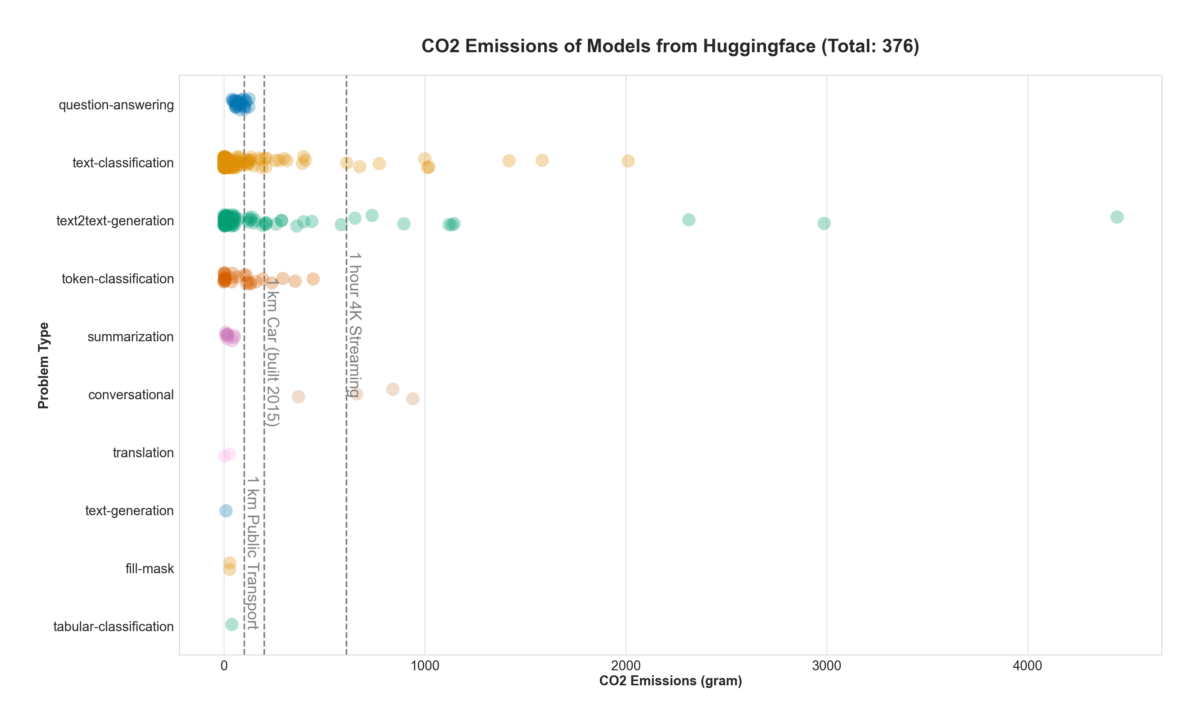
The experiment shows that the emissions for training a model are not always excessively high. Streaming in 4k, as an example, has a stronger impact than most of the above models. One might say that if you pass on the newest episode of Bridgerton today, you can train your Huggingface model with a clear conscience tomorrow.
However, the question is: who trained the models and where were they trained? Unfortunately, it is difficult to find out who exactly contributes to Huggingface. But this is crucial, since for our purposes only smaller projects matter. Finding out about the geographical location of the training is almost impossible, too. And depending on it, the emission can vary significantly because renewable energy is much more ecofriendly than fossil fuels. This makes it difficult to compare the model emissions directly.
How sustainable is Public Interest AI?
For the research project Public Interest AI, we develop AI prototypes that are designed to serve the public interest. For this post, we measured the electricity consumption for training these models. (Note that this is rather of anecdotal nature than being representative). Nevertheless, it should give some impressions about what dimension of CO2-emissions can be expected of small or medium sized machine learning projects.
The first prototype is intended to map (non-) accessible places. For this we deploy object detection, which automatically recognizes objects like stairs, steps, ramps, and stair-rails (Fig 3). For this task we previously annotated a dataset and chose YOLOv5 for the computer vision task. YOLOv5 is a widely used state-of-the-art deep neural network, and the technique for using it is called Transfer Learning: A pre-trained model is fine-tuned to our accessibility-dataset.
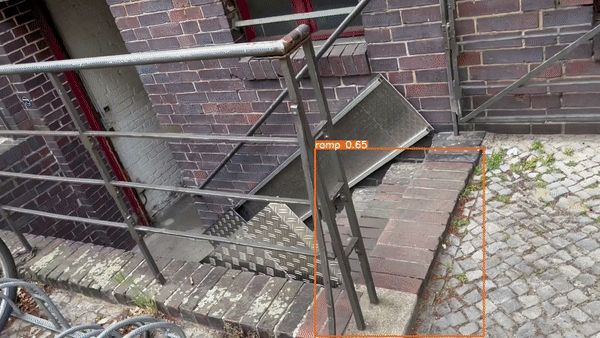
The second prototype is going to support fact-checkers in their work against disinformation. Before checking potential disinformation, one has to find a claim to check. The purpose of this natural language model is to spot such claims automatically to lower the workload of human fact-checkers.
For this task, we tried different machine learning models. First, we used “oldschool” models such as logistic regression and support vector machines, but we also used state-of-the-art models like an ensemble of transformers and a triplet network. These are instances of transfer learning, too.
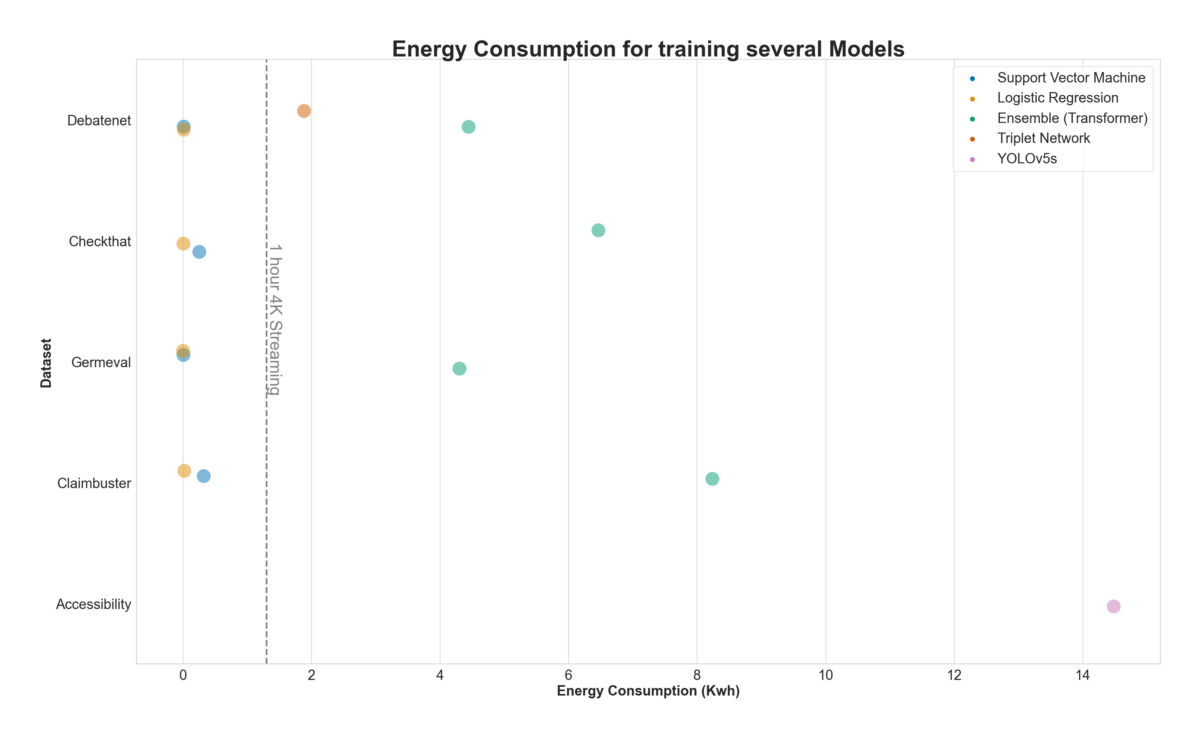
Fig 4 visualises the electricity consumption of the models. Two observations are central: The ensemble model has by far the highest electricity consumption. This is no surprise, since it is built from 60 individual transformers. On the other hand, it is striking that the electricity consumption for training these models is still only a little higher than Streaming one hour in 4k quality.
Where to train environmentally friendly?
As mentioned above, depending on the geographical location the same electricity consumption can emit different amounts of CO2. This is because of the given energy mix, and because of the fact that renewable energy emits less than fossil fuels. Fig 5 visualises the emissions of the ensemble model depending on the geographical location. Even though we trained in Germany, we can estimate the amount of CO2 that would have been emitted if we had trained in other countries.
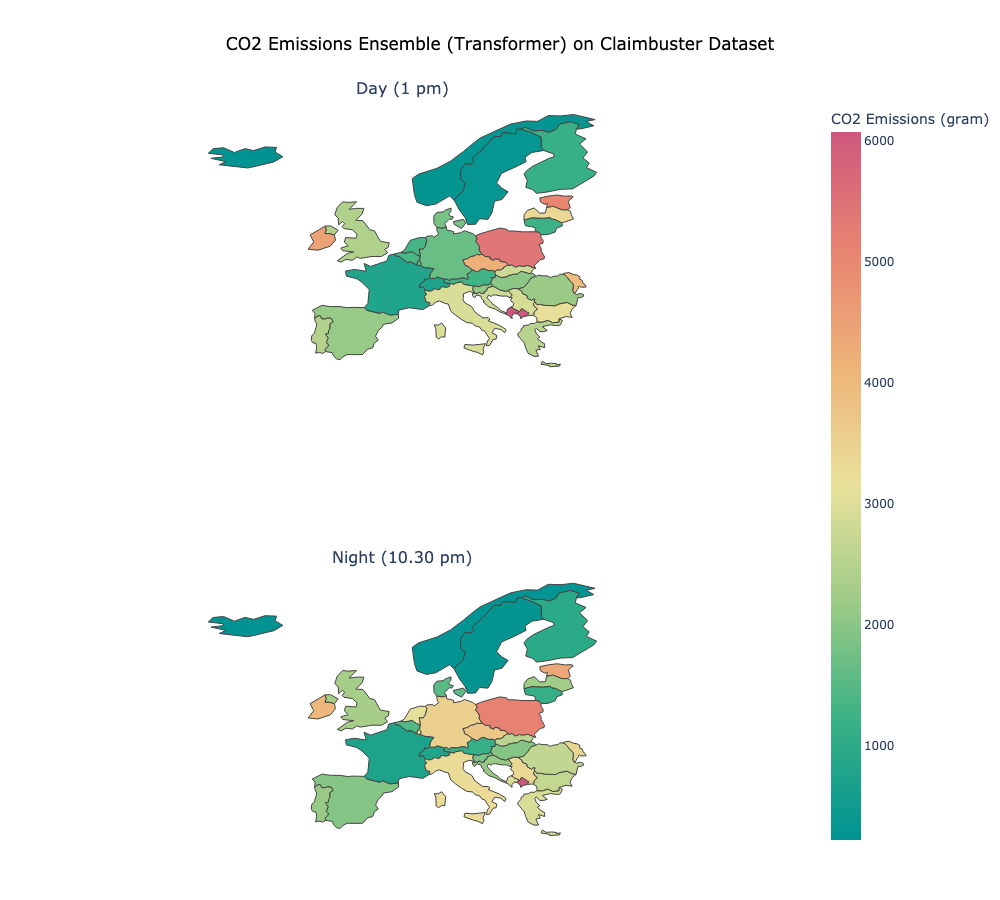
Fig 5 In which country and at what time emits training the most CO2? Emissions of an ensemble model of transformers trained on the Claimbuster dataset, split in day and night. The carbon intensity (gCO2/kWh) is taken from a sample from electricitymap.org on June 3rd 2022 (symbolic image).
The most striking observation is that the emission can be several times higher, depending on the geographical location, even though the electricity consumption stays the same. Furthermore, Fig 5 shows that in some countries it makes a huge difference if the training happens during daylight hours.
Recommendations and open questions
What can we learn from this and which questions remain unanswered? Most obviously, it turns out that small AI-Projects come with small emissions. This does not mean that there is no room for improvement. Nevertheless, it shows that the shocking numbers by Strubell et al cannot be generalised to all AI-projects.
On the other hand, it becomes clear that the data situation is not good. Neither the numbers from Huggingface nor our own experiments are representative. We still need more documentation. This is true for research as it is for real-world AI-projects. To be fair, during the process of writing we noticed that each week roughly 10 new models with proper documentation were added to Huggingface. It seems that sustainable AI is in fact becoming increasingly recognised. But documentation standards matter, too. As we saw earlier, emissions can vary significantly depending on the geographical location. Despite this fact, only in a few cases the location is documented. Details like this require further communication if we want to make sustainable AI reality.
Note
Code and data can be viewed on the authors’ Github repositories: https://github.com/SamiNenno/Sustainable-AI | https://github.com/rurfy/Sustainablity-AI
References
Ahmed, N., & Wahed, M. (2020). The De-democratization of AI: Deep Learning and the Compute Divide in Artificial Intelligence Research. ArXiv:2010.15581 [Cs]. http://arxiv.org/abs/2010.15581
Rohde, F., Wagner, J., Reinhard, P., Petschow, U., Meyer, A., Voß, M., & Mollen, A. (n.d.). Nachhaltigkeitskriterien für künstliche Intelligenz. Schriftenreihe Des IÖW, 220, 21.
Rolnick, D., Donti, P. L., Kaack, L. H., Kochanski, K., Lacoste, A., Sankaran, K., Ross, A. S., Milojevic-Dupont, N., Jaques, N., Waldman-Brown, A., Luccioni, A. S., Maharaj, T., Sherwin, E. D., Mukkavilli, S. K., Kording, K. P., Gomes, C. P., Ng, A. Y., Hassabis, D., Platt, J. C., … Bengio, Y. (2023). Tackling Climate Change with Machine Learning. ACM Computing Surveys, 55(2), 1–96. https://doi.org/10.1145/3485128
Strubell, E., Ganesh, A., & McCallum, A. (2019). Energy and Policy Considerations for Deep Learning in NLP. Proceedings of the 57th Annual Meeting of the Association for Computational Linguistics, 3645–3650. https://doi.org/10.18653/v1/P19-1355
van Wynsberghe, A. (2021). Sustainable AI: AI for sustainability and the sustainability of AI. AI and Ethics, 1(3), 213–218. https://doi.org/10.1007/s43681-021-00043-6
Dieser Beitrag spiegelt die Meinung der Autorinnen und Autoren und weder notwendigerweise noch ausschließlich die Meinung des Institutes wider. Für mehr Informationen zu den Inhalten dieser Beiträge und den assoziierten Forschungsprojekten kontaktieren Sie bitte info@hiig.de
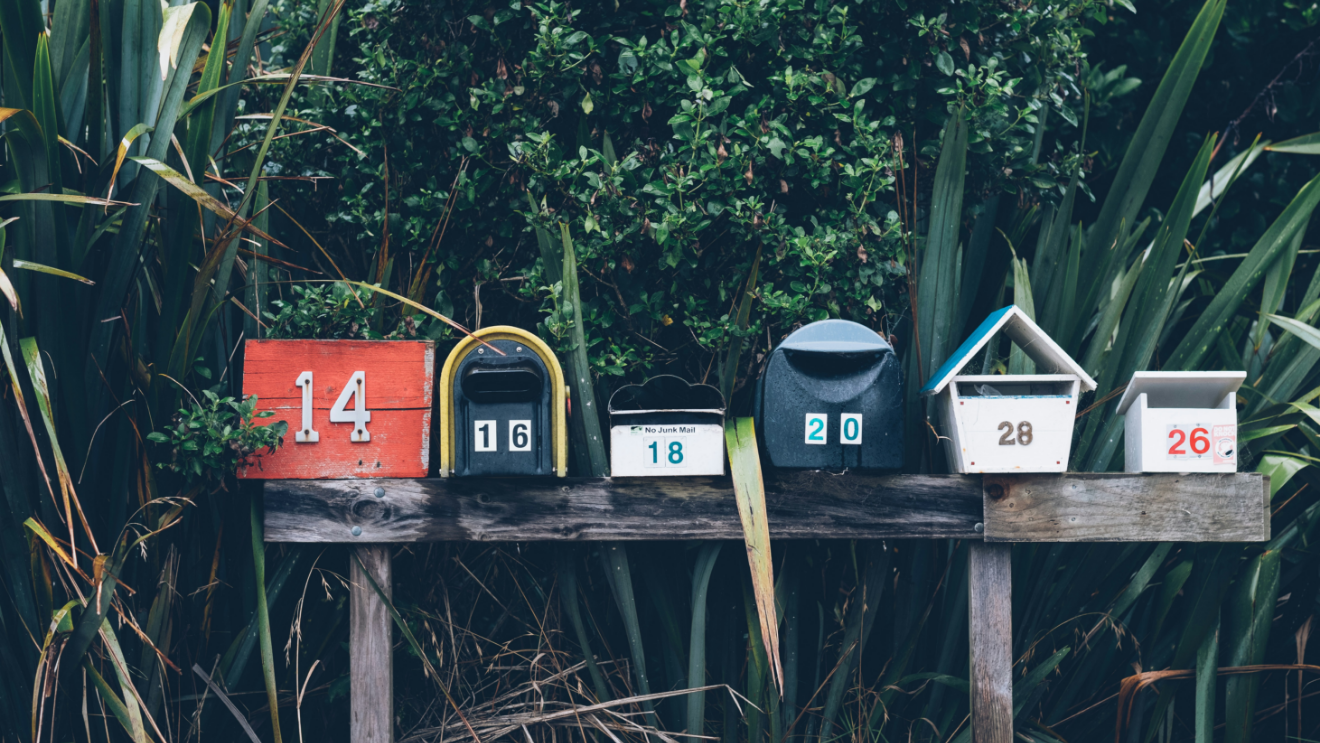
Jetzt anmelden und die neuesten Blogartikel einmal im Monat per Newsletter erhalten.
Digitalisierung und Nachhaltigkeit
Künstliche Intelligenz und Gesellschaft
Die Macht der Plattformen: Die Zukunft der Regulierung nach der Europawahl
Rückblickend auf die Europawahlen 2024 zieht dieser Beitrag Bilanz über die Auswirkungen des Gesetzes über digitale Dienste auf die Macht der Plattformen.
KI unter Aufsicht: Brauchen wir ‘Humans in the Loop’ in Automatisierungsprozessen?
Automatisierte Entscheidungen haben Vorteile, sind aber auch fehleranfällig. Ein Human in the Loop könnte helfen. Aber garantiert er bessere Ergebnisse?
Die Vielfalt von digitalen B2B-Plattformen
Dieser Blogbeitrag untersucht die Vielfalt digitaler Business-to-Business-Plattformen und kategorisiert sie nach Governance-Stilen und strategischen Zielen.