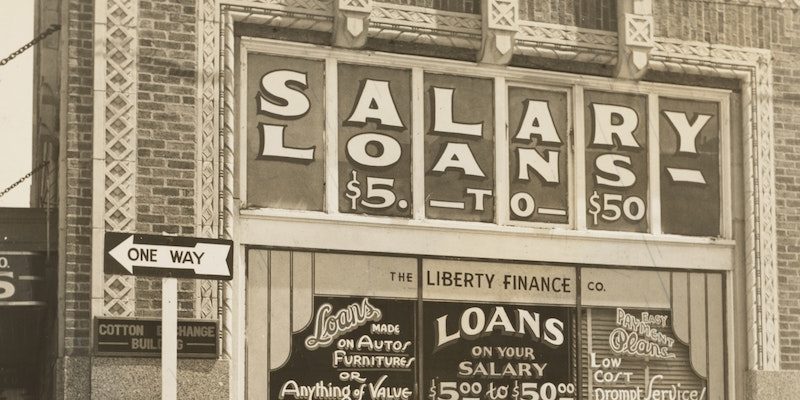
Alternative Kreditzugänge: Ein Vorteil für benachteiligte Personen?
ML-basierte Kreditscoring-Systeme könnten eine breitere finanzielle Inklusion auf dem Kreditmarkt ermöglichen. Dennoch droht die automatisierte Erstellung von Verbraucherprofilen finanzielle Ungleichheiten eher zu verstärken als zu beseitigen. Die Rechte, die Privatsphäre und die Autonomie der Verbraucher werden in einer schnelllebigen Online-Umgebung untergraben, die mit manipulativen UX-Mustern gespickt ist.
Consumer autonomy on the market for credit
In the blogpost 1 we argued that in the post-pandemic context, difficulties in accessing credit and financing digital tools may disconnect women from the increasingly digital society and curb their enjoyment of human rights. But is the consumer autonomy really that constrained, given the wide availability of online credit providers with a solution for every niche? Aren’t consumers opportunistic enough to compare what kind of data entries afford them with the most favourable credit terms? Isn’t the pool of people eligible for credit expanding with the benefit of using non-traditional credit scoring data alternative or complementary to SCHUFA?
Who is outsmarting whom?
The market is not short of solutions for people who do not have access to traditional bank credits or credit cards due to the lack of a steady income or a sufficient credit score. Almost all large online retailers offer financing options such as buy-now-pay-later solutions (e.g. Klarna) and instant consumer loans offered at the point of checkout (e.g. CreditClick). People are increasingly applying for smaller consumer loans online, which provides an avenue both for lenders and borrowers to overcome each others’ reservations. Similarly to buyers who learn to game online-retailers’ dynamic pricing practices, consumers may toy with online loan application forms, testing which type of “persona” or other data would lead to the best conditions. Such tinkering of credit applications may create the much-needed flexibility to accommodate applicants who do not fall seamlessly into any specific group, provided that they do not take on loans they cannot repay. However, consumers’ capacity to game the market for credit is nothing in comparison to the options available to creditors: they can employ manipulative web designs and engage consumer profiling on the basis of online behaviour and other data points.
Online credit options are designed to be applied with minimum effort. In the competition for consumers and the smoothest online experience, the service providers may be tempted to employ dark patterns: UX designs that nudge the user to make decisions they may not want to. Buy-now-pay-later solutions can entice people to take on financial commitments on many small amounts which upon accumulation may lead to over-indebtedness.
By law, consumer credit providers are obliged to inform the borrower of the nature of the agreement she is about to enter into in order to prevent overindebtedness. However, consumers are often insufficiently financially literate to understand the credit risks. Online retailers compete by offering the fastest and simplest check out experience. The length and language of mandatory information given at advertising and pre-contractual stages appears unsuitable for this fast-paced environment and therefore fails to protect consumers. Pre-ticked boxes and single-click availability of buy-now-pay-later loans may exploit behavioural biases to nudge vulnerable consumers into contracts that they may later regret due to the terms and conditions (e.g. higher interest rates). Ultimately, the webpages’ design is aimed to narrow the consumer’s freedom of choice – and freedom to tinker.
Scoring the unscorable
EU consumer credit laws oblige creditors to assess the creditworthiness of the consumer prior to extending credit based on information provided by the consumer and, where required, information from a database such as SCHUFA in Germany. Creditworthiness is defined by both credit risk to the lender and affordability for the borrower; therefore, it is in the interest of both parties. Besides mitigating the problems of asymmetric information and moral hazard with respect to borrowers, it also serves the public interest by preventing over-indebtedness on the part of consumers, ensuring the allocative efficiency and effective functioning of consumer credit markets.
Online credits use automated decision making systems in order to extend credit on the spot. Instant checkout credit solutions are data-driven and tend to infer credit risk from non-traditional/alternative data that are easily accessible in online environments. Many instant checkout loan providers exploit such alternative data which are taken as proxies for economic status, character and reputation. Address/zip code, occupation and social media data may impact the conditions under which credit is offered. In addition, machine learning algorithms may be used to derive your creditworthiness from your digital footprint, including the brand of your device, the email service provider you use, and the time of the day you shop. Vulnerability can also be inferred from such behavioural insights and exploited by lenders.
ML and big data based credit scoring uses larger datasets including alternative data points, increases the speed and lowers the cost of credit scoring. This is why it is suggested to foster financial inclusion of otherwise underserved or left out small, unscorable, invisible, and credit unworthy borrowers. However, due to problems of generalizability, inaccuracy and bias, there is, instead, a risk of discrimination and exclusion.
Is Discrimination 2.0 on the Horizon?
A consumer is often profiled when she starts exploring financing options. For instance, even the act of shopping around for the credit terms may influence the consumer’s chances of obtaining credit in the future, as every move may lead to an inference that affects the profile one ends up in. Such profiling is usually automated, and those being subject to it have little means to observe whether the credit option they are presented with reflects a potential bias. Machine learning algorithms can learn to associate creditworthiness with some behavioural patterns that are statistically observed more in the population of white men and discriminate against those who are not white and not men, perpetuating historic patterns of discrimination. In this case, information or behaviour associated with a group may become a proxy for creditworthiness which may curtail options for those who act differently. Another potentially discriminative problem occurs when available training data that was used to develop the ML model is not representative of all classes of borrowers and causes redlining of underrepresented groups which are more likely to consist of already disadvantaged groups.
Is the law ready for the challenge?
In most of these cases, consumers would not be aware that they had received a differentiated offer and were discriminated against compared to other groups of consumers which may or may not overlap with protected groups under anti-discrimination laws. Therefore, for example, even though discrimination based on sex, race and ethnicity in access to goods and services is prohibited under EU non-discrimination law, establishing a prima facie case of discrimination would be hard if not impossible. On the other hand, the groups created by machine learning algorithms may fall outside the scope of protected groups by law. Due to online profiling, even members of the same household may have dramatically different opportunities to access credit and obtain tools for maintaining their personal autonomy. In parallel, website designs do not support critical evaluation of the credit terms offered. Legal and policy solutions addressing access to credit, dark patterns, automated profiling must take into consideration their possible contribution to the structural discrimination of women and financially marginalized groups even when aiming at quite the contrary. It is up to debate whether GDPR, the first legislative piece to address algorithmic discrimination, offer the right tools to mitigate these risks.
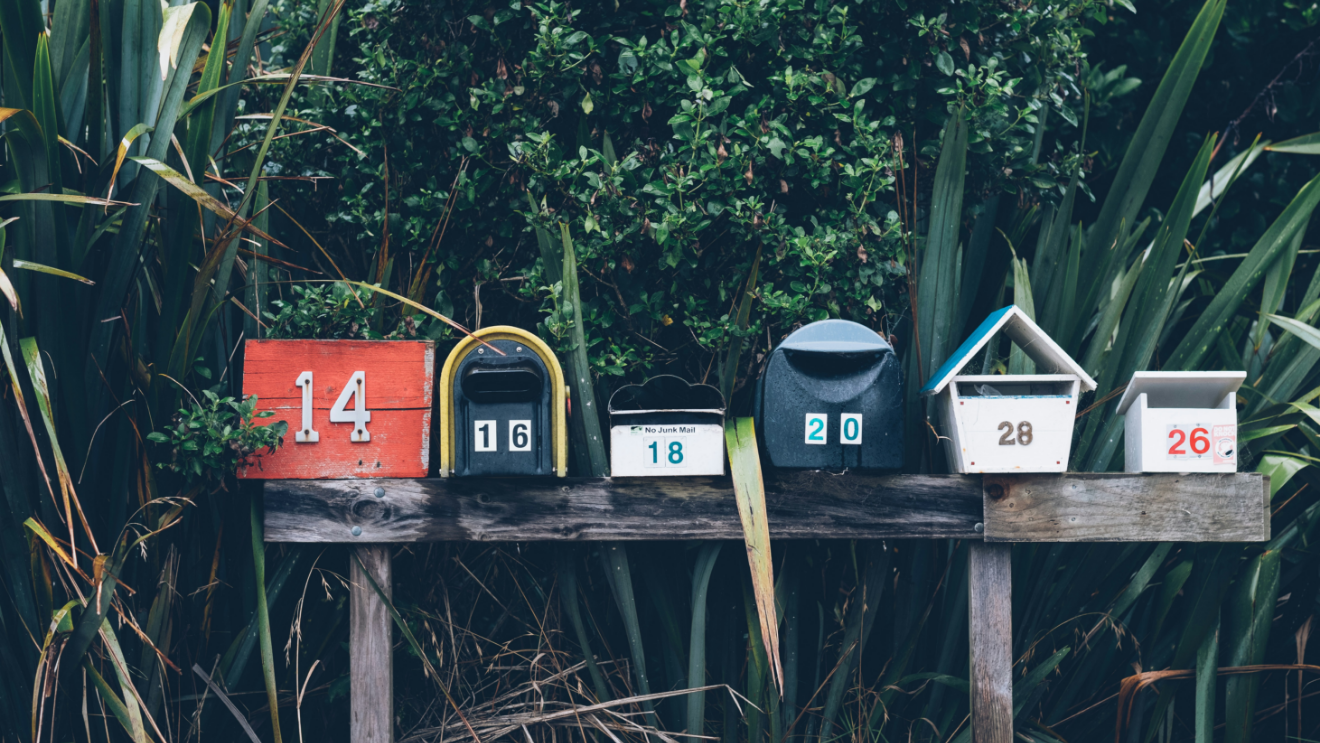
Jetzt anmelden und die neuesten Blogartikel einmal im Monat per Newsletter erhalten.
Data Governance
Beschäftigte durch Daten stärken
Arbeitsplätze werden zunehmend datafiziert. Doch wie können Beschäftigte und Gewerkschaften diese Daten nutzen, um ihre Rechte zu vertreten?
Zwei Jahre nach der Übernahme: Vier zentrale Änderungen im Regelwerk von X unter Musk
Der Artikel beschreibt vier zentrale Änderungen im Regelwerk der Plattform X seit Musks Übernahme 2022 und deren Einfluss auf die Moderation von Inhalten.
Zwischen Vision und Realität: Diskurse über nachhaltige KI in Deutschland
Der Artikel untersucht die Rolle von KI im Klimawandel. In Deutschland wächst die Besorgnis über ihre ökologischen Auswirkungen. Kann KI wirklich helfen?