Making sense of our connected world
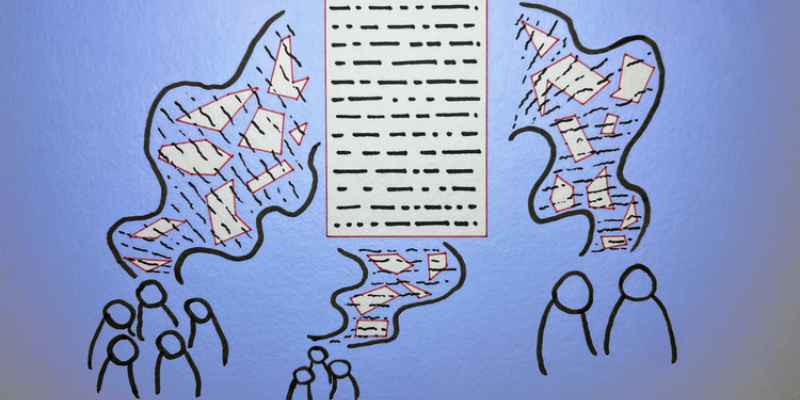
Empowering workers with data
Workers and their workplaces are becoming increasingly datafied. While much research has explored how this worker-related data is being used for management purposes, for example in the context of people analytics and algorithmic management, we know less about how workers and their representatives can leverage this data for their own purposes. Therefore, this blog post explores how some workers are organising around data to support worker advocacy.
The idea of using worker-related data to support worker advocacy is certainly not new. For example, circulating surveys among their members is a proven and well-tested method among unions around the world. In fact, Calacci (2022) argues that the very idea of a collective voice of workers assumes an understanding of workers’ collective desires, which is “clearly an information-driven practice” (p. 5). However, what we observe in workplaces today goes far beyond the production of data through means of simple surveys. Workers leave a variety of digital traces throughout their working day, which can be turned into data and analysed using increasingly complex and sometimes opaque methods (Leonardi, 2021).
How data reshapes the employment relationship
In workplace datafication, employee data typically flows from employees directly to their employers (Calacci, 2022). This can lead to data asymmetry, an information disadvantage, and power imbalances. It is most evident in but not limited to online labour platforms, where it has led to an increasing mobilisation against data-driven inequity (Kellogg et al., 2020). In traditional organisations, worker-related data is analysed to answer pressing management questions, such as whether a company will be affected by labour shortages or to identify reasons why employees quit. These analyses can provide meaningful insights and, in turn, inform management decisions. This process is referred to as people analytics.
Changing perspectives: Empowering workers with data
What about the pressing questions of workers and their representatives? To what extent can data be used to answer them? In an interview we conducted with a worker representative in 2021, she explains this potential as follows:
“I certainly see many opportunities […] that it may actually be possible to analyse data that we have access to in a different way. […] [So that] individual observations that you typically have, ‘someone is disadvantaged because they work part-time’ or ‘someone is disadvantaged because they are male or female […]’, can be backed up with […] a certain statistical value […]. [I]t always has a completely different effect when I can present figures, data and facts and say ‘here, look, out of […] 100 part-time employees, only one has actually managed […] to have a career’. […] I think that with […] clever data analyses and evaluations, […] you could certainly do worker representation in a different way.”
Similarly, in an Economist article of 2018, a representative of Sweden’s largest trade union explains how they envision that “algorithms could also predict the likelihood of lay-offs, if say a new chief executive takes over, and hence the need to get members ready to act”. As part of our project Between Autonomy and Surveillance, this led us to consider not only how we can design people analytics more worker-oriented (Köhne et al., 2024), but also how workers themselves can lead data-driven efforts.
Unlocking access to worker-related data
For unions and other bodies representing workers, access to information is crucial to their work (Calacci, 2022). So if they want to analyse novel sources of worker-related data—for example, to gain a better understanding of overwork dynamics—how can they gain access to it? We would like to outline two possible ways. First, they can request existing data from employers. For example, works councils can request reports or analyses on workforce diversity. This has to comply with data protection regulations and requires collaboration with employers. The challenge here is that this data, like all data, is curated and management has made decisions about what to collect and what to make visible (Calacci, 2022). Second, worker representatives can produce their own worker-related data. An example of this is the union surveys mentioned above, for example to gather workers’ experiences, opinions, and desires (Mateescu, 2023). While this allows for more control, it also requires significantly more resources.
Data-driven worker organising in practice
While many examples of data-driven worker organising come from the context of platform work, such as the Shipt Transparency Calculator and RooParse to improve pay transparency, some initiatives go beyond platform work. A widely cited example is WeClock, a self-tracking app that allows workers to record unpaid labour, untaken breaks, or job strain. Data is stored locally on workers’ devices but can be shared with unions and organisers. The Time Project is an anonymous data collection tool to tackle overwork in the television industry. Users can track how many (unpaid) hours they work per week and receive comparative data on hours and rates. Aggregated data provides evidence of overwork and can be used to advocate for better working conditions in the industry.
Exploring goals and requirements
In addition to the examples given above, there are certainly more to be found in grassroots movements as well as in traditional unions. These initiatives pursue various goals that include but are not limited to:
- Creating accountability by flagging workplace issues, for example through self-tracked data on well-being;
- challenging employer claims, for example through counter-data of time recordings;
- enhancing bargaining position, for example through aggregated data on competencies and expected shortages;
- building solidarity and attracting members, for example by mapping shared experiences.
In a recent workshop we organised for worker representatives, it became evident that many participants see the potential of using data to answer questions relevant to workers, such as pay transparency, strategic personnel planning, time data related to remote working, people development or workplace equality.
However, the resources required are seen as a significant challenge. Similarly, an analysis of union responses to scientific management in the mid-20th century found that while unions demanded data transparency (“show me” approach) and circulated wage surveys, they were rather sceptical about conducting their own time studies because “it was tedious, expensive, and required technical expertise, to which few unions had regular access” (Khovanskaya et al., 2019, p. 12). Advocating for workers’ interests with data requires, among other things, data literacy, technical infrastructure and approaches to secure data governance (Nyman et al., 2024). As with employer-led people analytics, the data itself is not inherently useful; analysing it and making it actionable requires extensive resources. According to our workshop participants, they currently face two key challenges. First, they often lack awareness of the data, systems and methods available to develop their own use cases. Second, they often struggle to gain access to existing data from management. Discussions during the workshop highlighted the need to showcase best practices and use cases that can generate value for worker representatives.
Towards worker-led data projects
While data can provide meaningful insights into today’s workplaces, it matters who has control over this data. Worker data itself is not neutral, but rather political, and its meaning can be contested. Data analytics is a means of producing knowledge about the workplace and it is crucial that workers participate in the production of this knowledge. The interests of those leading people analytics efforts are directly and indirectly inscribed in questions, tools, or metrics. Our project has provided insights into how people analytics can be designed in a more worker-oriented way (Köhne et al., 2024). However, from the perspective of workers, this potential worker orientation has limitations because—despite the data-driven promise—people analytics processes are characterised by human judgement and decision-making. We therefore hope that future research will increasingly consider the datafication of the workplace not only from the perspective of managers but also from the perspective of workers and how they use data to achieve their own goals. To this end, researchers could build on ongoing debates around “worker data science” (Gallagher et al., 2023), “digital workerism” (Woodcock, 2021), and “data-driven labour organizing” (Nyman et al., 2024). This raises the question of not simply countering management’s people analytics but developing productive ways of working together to use data in ways that benefit both management and workers. Answering this question requires more research in this area but also funding for the development of practical tools and insights.
References
Calacci, D. (2022). Organizing in the end of employment: Information sharing, data stewardship, and digital workerism. 2022 Symposium on Human-Computer Interaction for Work, 1–9. https://doi.org/10.1145/3533406.3533424
Technology may help to revive organised labour. (15.11.2018). The Economist. https://www.economist.com/briefing/2018/11/15/technology-may-help-to-revive-organised-labour
Gallagher, C., Gregory, K., & Karabaliev, B. (2023). Digital worker inquiry and the critical potential of participatory worker data science for on‐demand platform workers. New Technology, Work and Employment, 1-21. https://doi.org/10.1111/ntwe.12286
Kellogg, K. C., Valentine, M. A., & Christin, A. (2020). Algorithms at work: The new contested terrain of control. Academy of Management Annals, 14(1), 366–410. https://doi.org/10.5465/annals.2018.0174
Khovanskaya, V., Dombrowski, L., Rzeszotarski, J., & Sengers, P. (2019). The tools of management: Adapting historical union tactics to platform-mediated labor. Proceedings of the ACM on Human-Computer Interaction, 3(CSCW), 1–22. https://doi.org/10.1145/3359310
Köhne, S., Klöpper, M., von Richthofen, G., & Send, H. (2024). Autonomer dank Algorithmen? People Analytics aus Perspektive der Selbstbestimmung. WSI-Mitteilungen, 77(1), 18–25. https://doi.org/10.5771/0342-300X-2024-1-18
Leonardi, P. M. (2021). COVID‐19 and the new technologies of organizing: Digital exhaust, digital footprints, and artificial intelligence in the wake of remote work. Journal of Management Studies, 58(1), 249–253. https://doi.org/10.1111/joms.12648
Mateescu, A. (2023). Explainer: Challenging worker datafication. Data & Society.
Nyman, S., Benfeldt, O., Gierlich-Joas, M., Bagger, C., Zambach, S., Blicher, A., Mazumdar, S., & Nã, J. (2024). From algorithmic management to data- driven labour organising. A trade union approach to workplace datafication. Scandinavian Journal of Information Systems, 13-38.
Woodcock, J. (2021). Towards a digital workerism: Workers’ inquiry, methods, and technologies. NanoEthics, 15(1), 87–98. https://doi.org/10.1007/s11569-021-00384-w
Image credits
Yasmine Boudiaf & LOTI / Better Images of AI / Data Processing / CC-BY 4.0. https://betterimagesofai.org/images?artist=YasmineBoudiaf&title=DataProcessing
This post represents the view of the author and does not necessarily represent the view of the institute itself. For more information about the topics of these articles and associated research projects, please contact info@hiig.de.
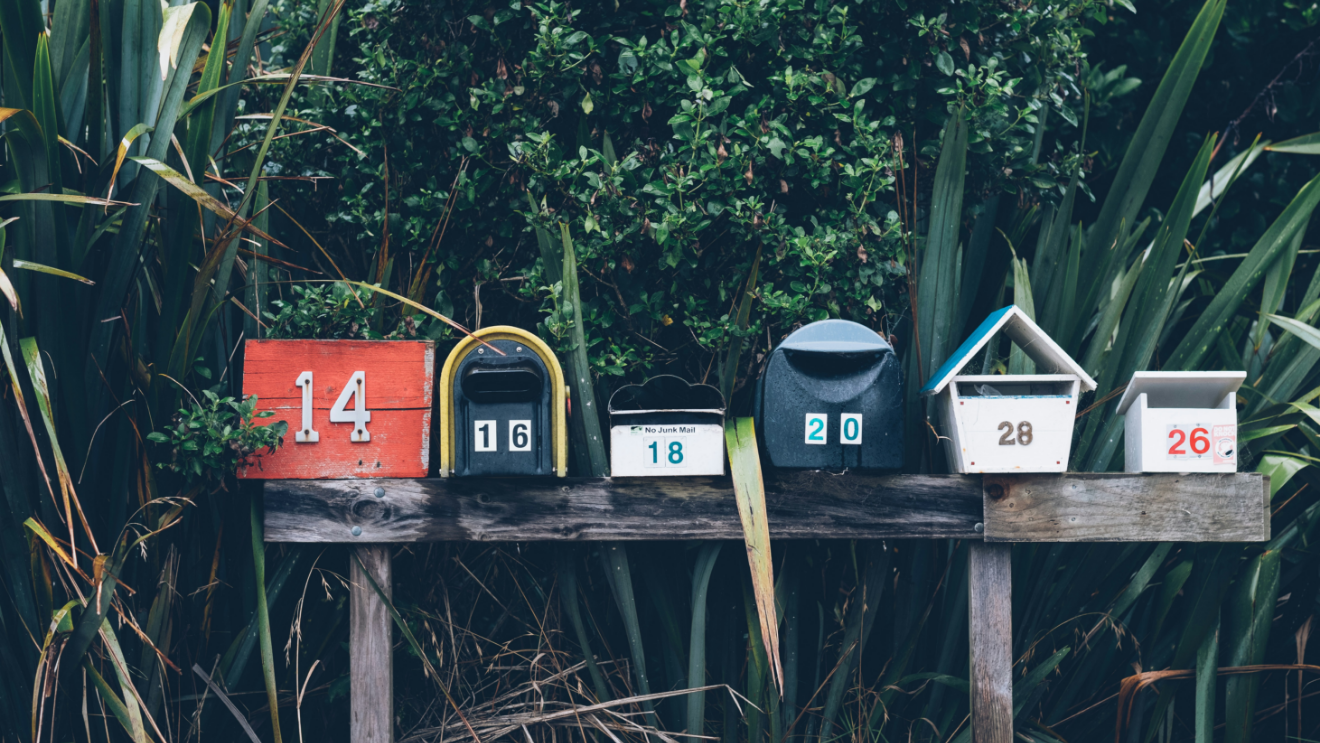
You will receive our latest blog articles once a month in a newsletter.
Digital future of the workplace
AI at the microphone: The voice of the future?
From synthesising voices and generating entire episodes, AI is transforming digital audio. Explore the opportunities and challenges of AI at the microphone.
Do Community Notes have a party preference?
This article explores whether Community Notes effectively combat disinformation or mirror political biases, analysing distribution and rating patterns.
How People Analytics can affect the perception of fairness in the workplace
People Analytics in the workplace can improve decisions but may also heighten feelings of unfairness, impacting employee trust and workplace relationships.