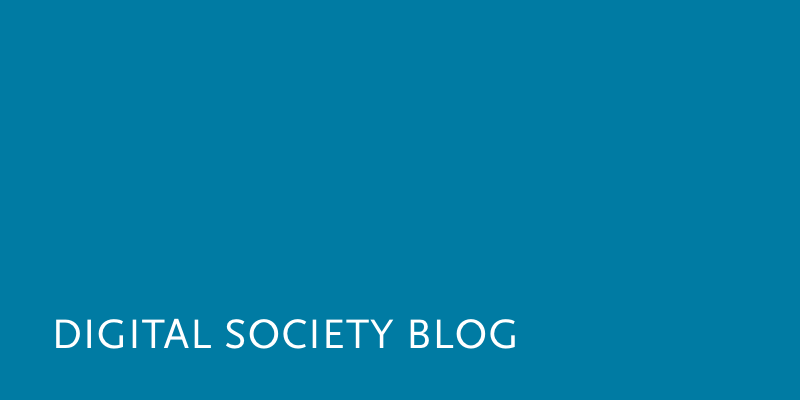
Profiling the unemployed
Since 2014, job centres in Poland have been using an algorithmic decision-making system to categorize unemployed people and allocated different types of assistance. In the context of the global financial crisis in 2007, job centres have tried to become more efficient, among other things by introducing more individual advice by profiling the unemployed using an algorithm. However, this new tool created many challenges for a transparent decision-making and effective use of social rights. In this article, five lessons learned from profiling the unemployed are presented as a case of automated decisions in public administration.
Process of categorization
Profiling mechanism is a scoring system aiming to divide unemployed people into three categories. The system is based on processing personal data, which is collected through a survey and an interview – a total of 24 data points. Each of them is assigned with specific volume of scores (0-8). Eight data points are collected during the registration at the job centre – for example, age, gender, disability, knowledge of foreign languages or duration of unemployment. Another 15 data points are gathered during the computer-based interview. The questions are constructed in a way that suggests that they are open-ended in character. But in reality, the scope of the answers is closed. For example, the question “What is the main obstacle for returning to the job market” has 22 predetermined answers. Based on the final score an algorithm decided which category should be given to the unemployed. The final calculation determines the scope of assistance which a person can apply for. According to statistics profile I covers 2% of the unemployed in Poland, profile II – 65%, and profile III – 33%.
More articles about algorithmic decisions and human rights
Social and legal implications
From the beginning, this profiling mechanism caused many confusions among clerks and unemployed people. Citizens applying for the assistance at job centres didn’t know the nature of this mechanism and its outcomes. In many cases assigned profiles were treated as blockades for receiving specific types of assistance. However the rules were constructed that it was difficult to change a person’s category. In practice, unemployed persons were trying to game the system in various different ways.
Polish Data Protection Authority, the Ombudsman, civil society organizations and trade unions were raising concerns over lack of specific safeguards for unemployed and imprecise scope of processed data. Now even the Polish government admits that there are some problems. The Ministry of Family, Labour and Social Policy recently informed that the government is planning to change the mechanism. However, no draft proposal was presented to the public yet. Meanwhile the Ombudsman referred profiling case to the Constitutional Court.
Further lessons
The profiling case raises few important lessons for introducing automated or semi-automated decision-making systems in public administration:
- The translation of the law into a code must be subject to democratic control. One of the biggest problems in the profiling case was an inaccuracy between the legal text and the IT systems functionalities (e.g. the scope of processed data or rules of changing the assigned profile). Policy makers should pay attention how system developers are translating legal provision into the code. Creating new automated-decision making systems in public administration could affect thousands of people in the standardize way and at the end lead to creation of new legal norms. This translation process should be subject to detailed supervision by the relevant institutions like courts, regulators or special parliamentary commission.
- People are using technologies in different ways. Initially, this profiling mechanism was designed to be only an advisory tool – it was a clerk who should make final decision about the profile. However research has shown that clerks in job centers apply differentiated strategies for using profiling mechanism. For many, the computer was a definitive decision-maker. For others, profiling was just a part of the broader diagnosis process. Another strategy was adjusting the profile to the expectations of the unemployed person. These examples show that in practice, the use of automated technologies depends on institutional issues, competencies and individual preferences. Designers’ intentions may be significantly different from the actual use of technology, and the level of automatization results not only from initial assumptions, but above all from the practice of users.
- Transparency and other safeguards are crucial. The process of profiling plays crucial role in shaping the unemployed situation. However the logic behind the profiling and the algorithm itself were treated as confidential information. As a result, the unemployed didn’t know how certain individual features or life circumstances affect his chance of being assigned to a given category. The questions which are asked during the interview with the unemployed became public only after an intervention from the Panoptykon Foundation. Scoring rules were published after the court’s judgment. This case shows that a person subjected to automated decisions should have a legal right to obtain detailed information about all aspects of this process that might affect her situation (including the logic behind it, what data was used and with what result etc.), as well as to be offered human intervention and explanation of the final result.
- Courts and human rights institutions must learn how to work with algorithms. Profiling the unemployed was subject of intervention from the Ombudsman, Data Protection Authority and courts. The scope and level of these interventions vary. However, this examples show that the technological layer is a problem in assessing the consequences for human rights. In such situations, the institutions should cooperate and exchange their experiences (e.g. DPA in the technical aspects of data processing, and the Ombudsman in the context of discrimination). We also have to consider whether algorithms used in the process of decision-making by public administration shouldn’t be subjected to prior approval of independent bodies capable of evaluating human rights-related risks.
- Not all decisions should be automatized. In the pursuit of increasing the efficiency of public administration through automatization, one should ask which processes can be automated and which should not. Elements that should be taken into account should be the scope of discretion, rule of law principles, potentially negative effects on citizens and the consequences for human rights.
Jędrzej Niklas has a PhD in international law. He is currently working at Department of Media and Communications at London School of Economics (LSE) and is a fellow at Centre for Internet & Human Rights, European University Viadrina. His research focuses on the relations between human rights and data-driven technologies. This article is based on the report Profiling the Unemployed in Poland: Social And Political Implications Of Algorithmic Decision Making.
The article above is part of a series on algorithmic decisions and human rights. If you are interested in submitting an article yourself, send us an email with your suggestions.
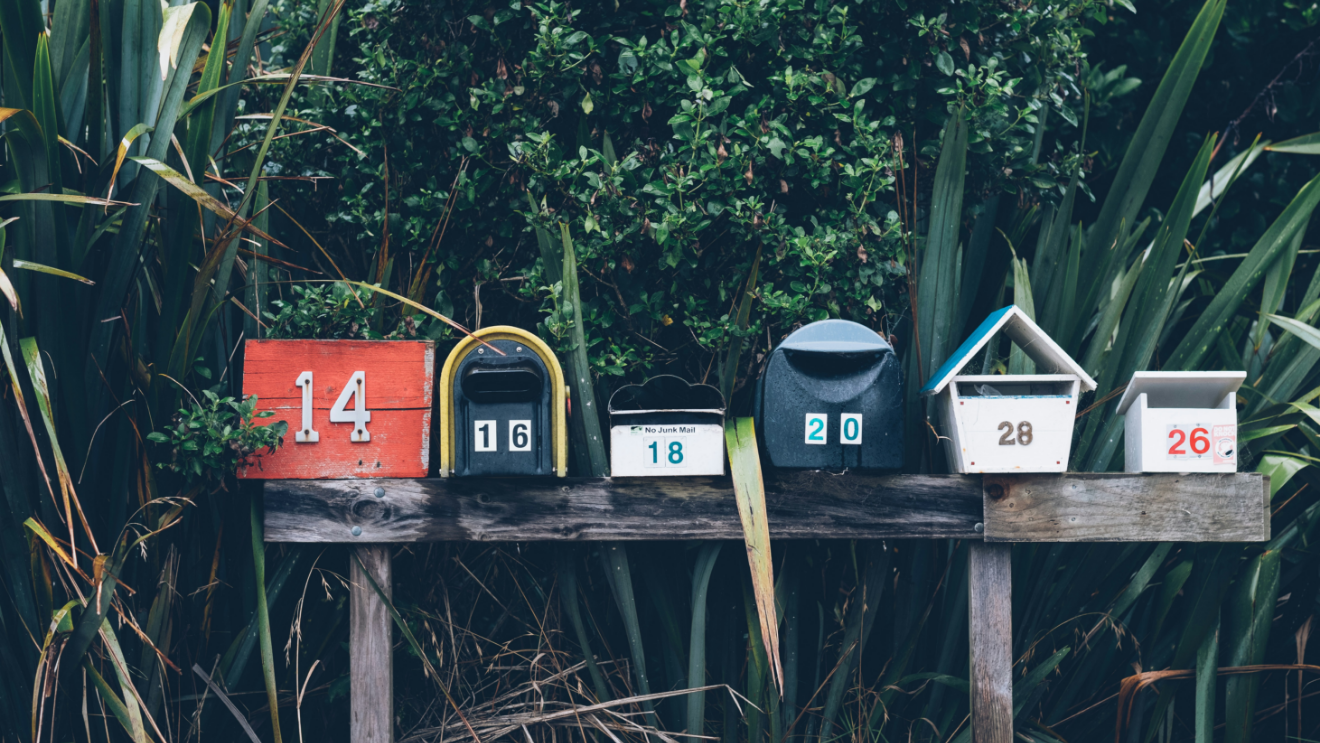
You will receive our latest blog articles once a month in a newsletter.
Research issues in focus
Escaping the digitalisation backlog: data governance puts cities and municipalities in the digital fast lane
The Data Governance Guide empowers cities to develop data-driven services that serve citizens effectively.
Online echoes: the Tagesschau in Einfacher Sprache
How is the Tagesschau in Einfacher Sprache perceived? This analysis of Reddit comments reveals how the new simplified format news is discussed online.
Opportunities to combat loneliness: How care facilities are connecting neighborhoods
Can digital tools help combat loneliness in old age? Care facilities are rethinking their role as inclusive, connected places in the community.