Making sense of our connected world
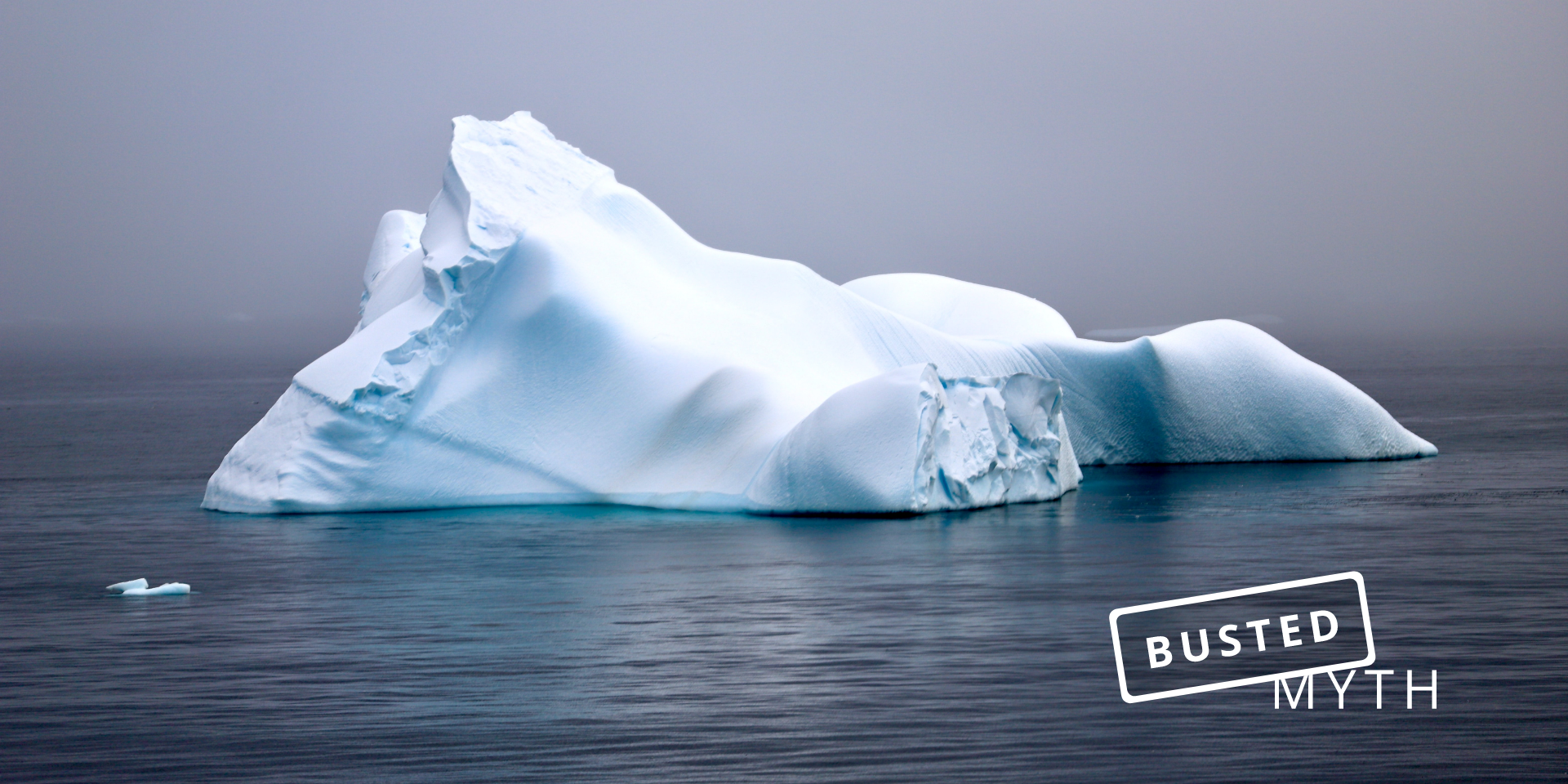
Myth: AI will save us from climate change
AI provides powerful tools to tackle climate change in various applications – but it is not a silver bullet. It can support the mitigation of climate change, for instance, by helping reduce greenhouse gas emissions within various applications. It can support adapting to a changing climate. AI can even support climate science itself. However, it can also be used to harm the climate. To avoid that, AI applications should be developed in collaboration and ongoing exchange with the communities that will use or are otherwise affected by the technology to avoid unforeseen impacts and drawbacks.
Mythos
AI will save us from climate change.
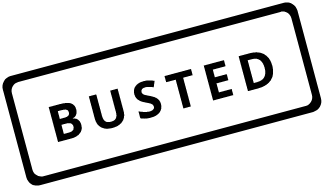
AI alone will not save us from climate change! If applied in the wrong areas, it can even harm the climate. However, AI provides powerful tools to address climate change in various applications.
Watch the talk
Materials
Presentation slides | |
KEY LITERATURE Kaack, L., Donti, P., Strubell, E. & Rolnick, D. (2020). Artificial Intelligence and Climate Change. Opportunities, considerations, and policy levers to align AI with climate change goals. Rolnick, D., Donti, P., Kaack, L., Kochanski, K., Lacoste, A., Sankaran, K., Ross, A., Milojevic-Dupont, N., Jaques, N., Waldman-Brown, A., Luccioni, A., Maharaj, T., Sherwin, E., Mukkavilli, S., Kording, K., Gomes, C., Ng, A., Hassabis, D., Platt, J., Creutzig, F., Chayes, J. & Bengio, Y. (2019). Tackling Climate Change with Machine Learning. ADDITIONAL READINGS Battiston, S., Mandel, A., Monasterolo, I., Schütze, F. & Visentin, G. (2017). A climate stress-test of the financial system. Nature climate change, 7 (4), 283–288. BP. (2020). Statistical Review of World Energy. Carleton, T. A. & Hsiang, S. M. (2016). Social and economic impacts of climate. Science, 353 (6304), aad9837–aad9837. Carleton, T. & Greenstone, M. (2021). Updating the United States Government’s Social Cost of Carbon. University of Chicago, Becker Friedman Institute for Economics Working Paper No. 2021-04, Available at SSRN Electronic Journal. Friedlingstein, P., Jones, M. W., O’Sullivan, M., Andrew, R. M., Hauck, J., Peters, G. P., Peters, W., Pongratz, J., Sitch, S., Le Quéré, C., Bakker, D. C. E., Canadell, J. G., Ciais, P., Jackson, R. B., Anthoni, P., Barbero, L., Bastos, A., Bastrikov, V., Becker, M., Bopp, L., Buitenhuis, E., Chandra, N., Chevallier, F., Chini, L. P., Currie, K. I., Feely, R. A., Gehlen, M., Gilfillan, D., Gkritzalis, T., Goll, D. S., Gruber, N., Gutekunst, S., Harris, I., Haverd, V., Houghton, R. A., Hurtt, G., Ilyina, T., Jain, A. K., Joetzjer, E., Kaplan, J. O., Kato, E., Goldewijk, K. K., Korsbakken, J. I., Landschützer, P., Lauvset, S. K., Lefèvre, N., Lenton, A., Lienert, S., Lombardozzi, D., Marland, G., McGuire, P. C., Melton, J. R., Metzl, N., Munro, D. R., Nabel, J. E. M. S., Nakaoka, S.-I., Neill, C., Omar, A. M., Ono, T., Peregon, A., Pierrot, D., Poulter, B., Rehder G., Resplandy, L., Robertson, E., Rödenbeck, C., Séférian, R., Schwinger, J., Smith, N., Tans, P. P., Tian, H., Tilbrook, B., Tubiello, F. N., van der Werf, G. R., Wiltshire, A. J. & Zaehle, S. (2019). Global Carbon Budget 2019, Earth System Science Data, 11 (4), 1783–1838. Hsu, A. & Rauber, R. (2021). Diverse climate actors show limited coordination in a large-scale text analysis of strategy documents. Communications Earth & Environment, 2 (30), 1–12. IPCC. (2013). Summary for Policymakers. In: Climate Change 2013: The Physical Science Basis. Contribution of Working Group I to the Fifth Assessment Report of the Intergovernmental Panel on Climate Change. [Stocker, T.F., Qin, D., Plattner, G.-K., Tignor, M., Allen, S.K., Boschung, J., Nauels, A., Xia, Y., Bex, V. & Midgley, P.M. (eds.)]. IPPC. (2018). Global Warming of 1.5 ºC. An IPCC Special Report on the impacts of global warming of 1.5°C above pre-industrial levels and related global greenhouse gas emission pathways, in the context of strengthening the global response to the threat of climate change, sustainable development, and efforts to eradicate poverty. [Masson-Delmotte, V., Zhai, P., Pörtner, H.-O., Roberts, D., Skea, J., Shukla, P.R., Pirani, A., Moufouma-Okia, W., Péan, C., Pidcock, R., Connors, S., Matthews, J.B.R., Chen, Y., Zhou, X., Gomis, M.I., Lonnoy, E., Maycock, T., Tignor, M. & Waterfield, T. (eds.)]. Klusak, P., Agarwala, M., Burke, M., Kraemer, M. & Mohaddes, K. (2021). Rising temperatures, falling ratings: The effect of climate change on sovereign creditworthiness. CAMA Working Paper No. 34/2021, Available at SSRN. NOAA National Centers for Environmental Information. (2020). State of the Climate: Global Climate Report for Annual 2019. Nguyen, V. N., Jenssen, R. & Roverso, D. (2018). Automatic autonomous vision-based power line inspection: A review of current status and the potential role of deep learning. International Journal of Electrical Power & Energy Systems, 99, 107-120. Strubell, E., Ganesh, A. & McCallum, A. (2019). Energy and Policy Considerations for Deep Learning in NLP. ArXiv:1906.02243 [Cs]. Voss, M., Heinekamp, J. F., Krutzsch, S., Sick, F., Albayrak, S. & Strunz, K. (2021). Generalized Additive Modeling of Building Inertia Thermal Energy Storage for Integration Into Smart Grid Control. IEEE Access, 9, 71699-71711. World Greenhouse Gas Emissions: 2016. (o. D.). World Resources Institute. Abgerufen am 10. Juni 2021. Yu, J., Wang, Z., Majumdar, A. & Rajagopal, R. (2018). DeepSolar: A machine learning framework to efficiently construct a solar deployment database in the United States. Joule, 2(12), 2605-2617. | |
UNICORN IN THE FIELD Climate Change AI Future Changers – Der Podcast für nachhaltige Innovation |
About the authors
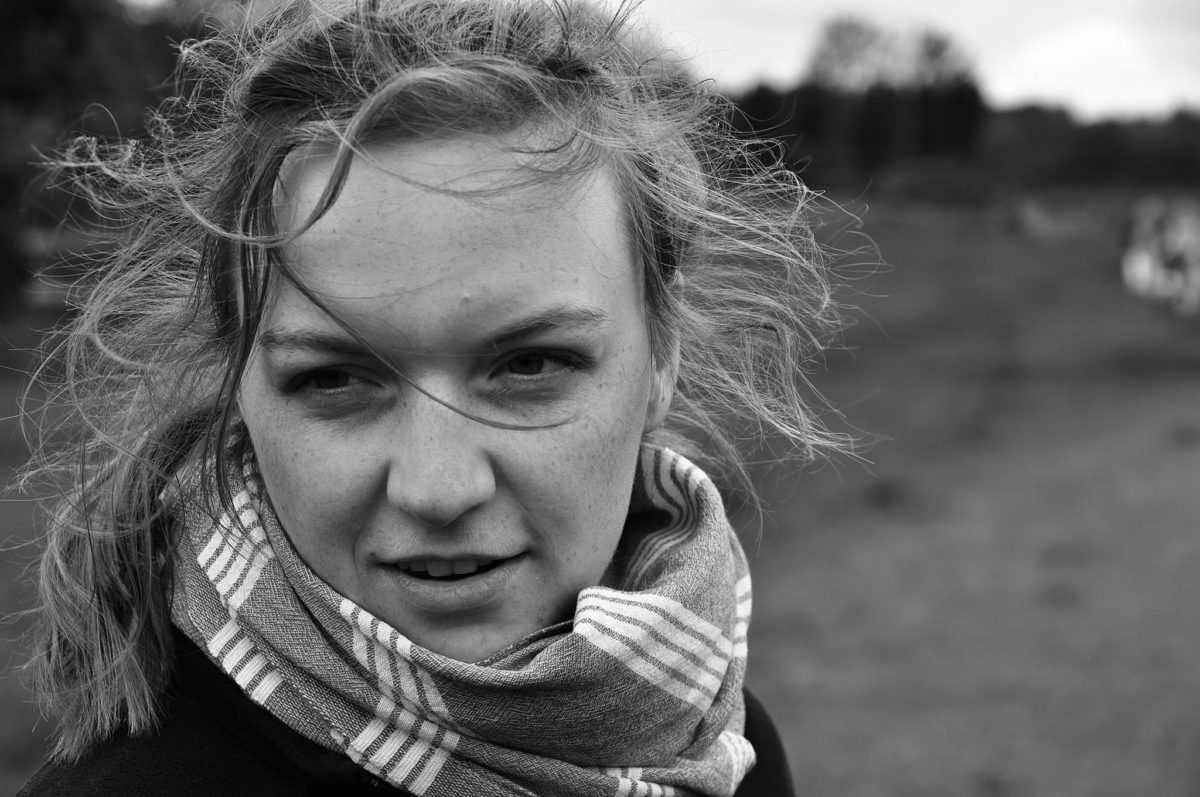
Raphaela Kotsch
Raphaela is a PhD student in Political Economy and Development at the University of Zurich and holds an M.Sc in Environmental Economics from the London School of Economics. She works at the intersection of climate policy, environmental economics and machine learning. As part of her fellowship with the Digitalization Initiative of the Zurich Higher Education Institutions (DIZH), she works on leveraging AI methods to increase trust and transparency in carbon markets. As the community lead for economics and markets at ClimateChangeAI, she bridges between economics, the computational and climate social sciences.
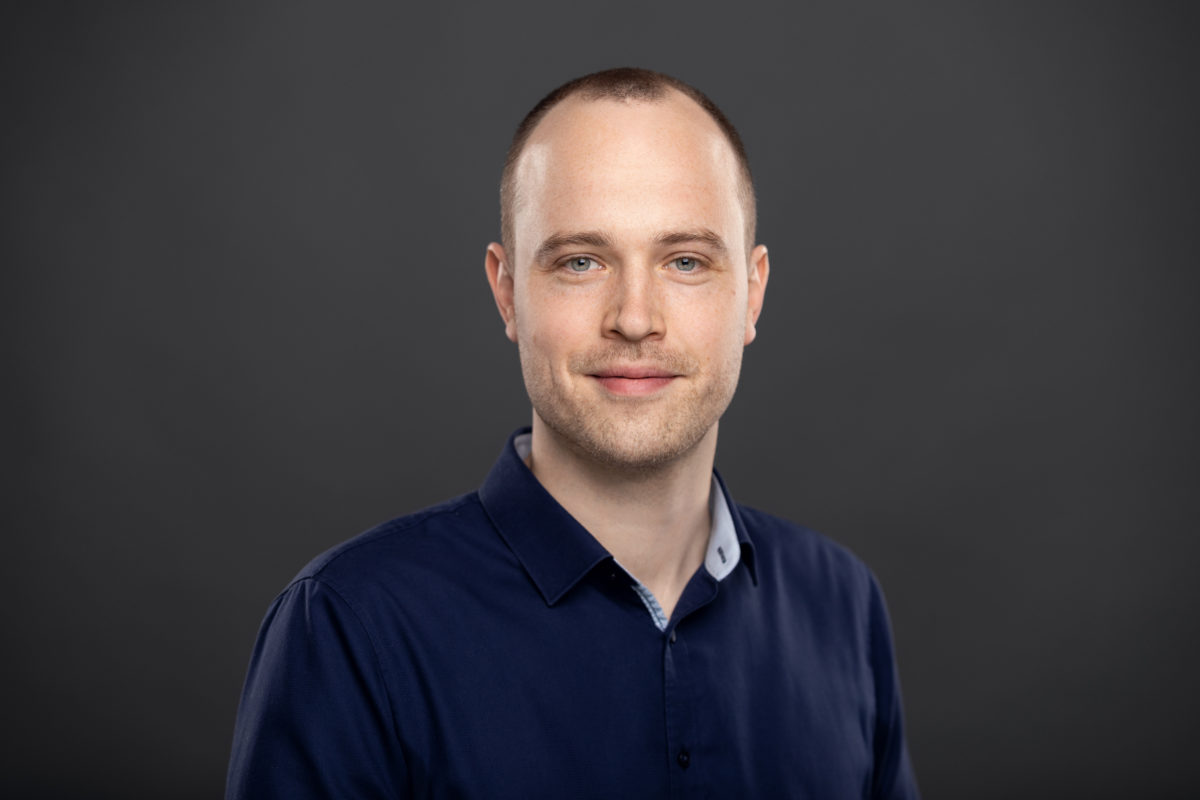
Marcus Voß
Marcus is a PhD student at TU Berlin, where he heads the research group Smart Energy Systems at the DAI-Lab. There he has worked on several research projects investigating how digitalization and AI can support the energy transition. In the project “SustAIn: Sustainability Index for Artificial Intelligence”, he studies how AI-systems can be implemented more sustainably. He received his M.Sc. degree from the Humboldt University of Berlin. At ClimateChangeAI, he provides content and resources to enable people who want to start researching, teaching, and working on the intersection of AI and climate change.
Why, AI?
This post is part of our project “Why, AI?”. It is a learning space which helps you to find out more about the myths and truths surrounding automation, algorithms, society and ourselves. It is continuously being filled with new contributions.
This post represents the view of the author and does not necessarily represent the view of the institute itself. For more information about the topics of these articles and associated research projects, please contact info@hiig.de.
Artificial intelligence and society
Sign up for HIIG's Monthly Digest
and receive our latest blog articles.
Debunking Science Myths: Preconceptions about science put to the test
What is really true about preconceptions about science? Four popular myths about a constantly quarrelling group of professionals explained simply.
No Smartphone = Cringe Weirdo
In this blog post, author Jascha Bareis shares his experiences since getting his first smartphone just this year.
European elections and digital policy: German party positions
To what extent are German parties addressing digital policy in the European elections? A glance at the election programmes reveals different priorities.