Making sense of our connected world
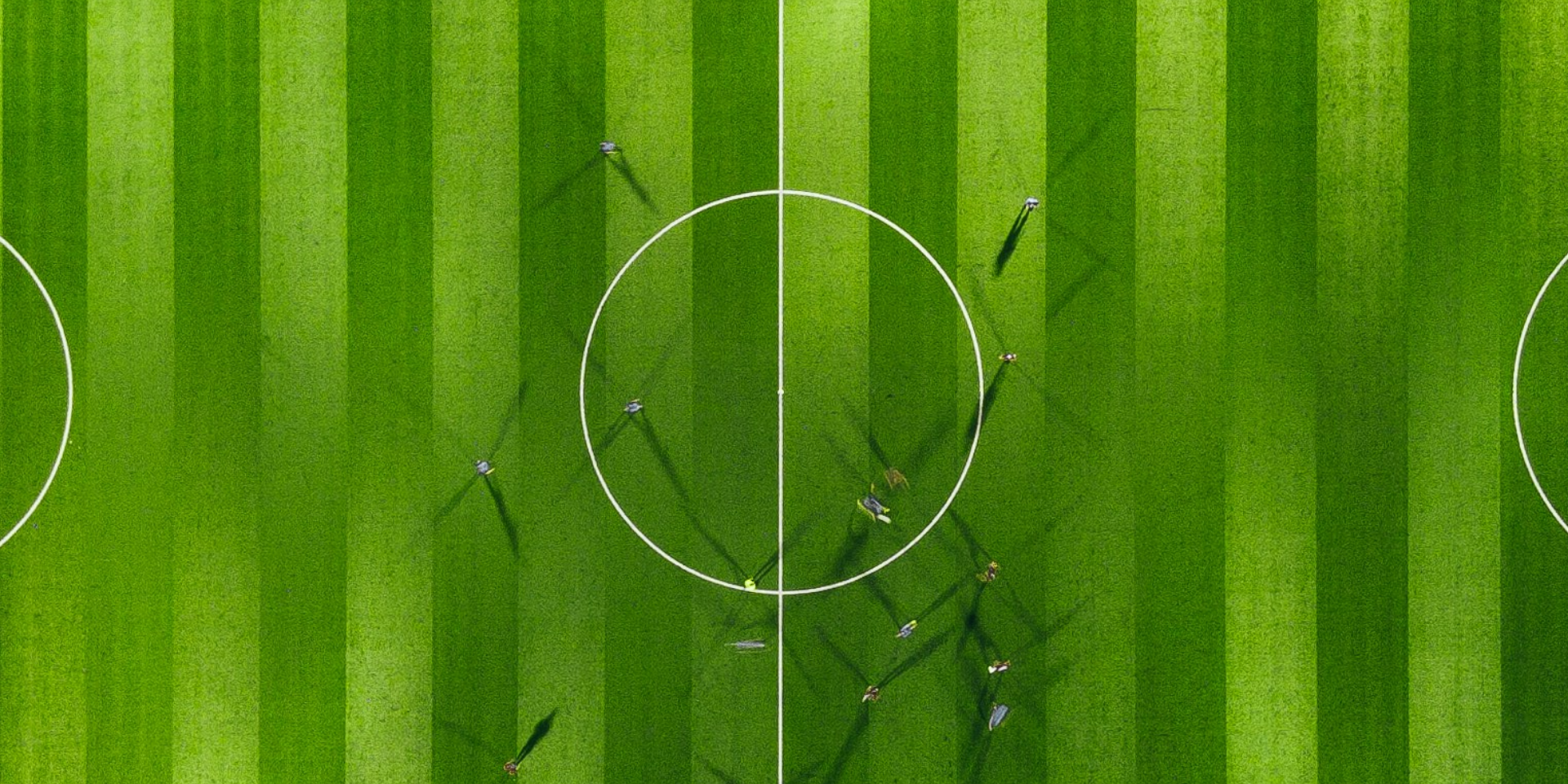
AI under supervision: Do we need Humans in the Loop in automation processes?
Who is this human that is kept in the loop? In the era of artificial intelligence, vast amounts of data are processed to generate automated recommendations and even make decisions. These systems are increasingly integral to our daily lives: for instance, automated systems in banks assess the creditworthiness of potential applicants, while aiding doctors in diagnosing illnesses. Thus, the benefits of automation seem clear to many. On the one hand, they accelerate numerous work processes and operations, with the aim to reduce costs. On the other hand, AI-driven systems could identify hidden relationships and patterns that humans may overlook. However, despite their advantages, automated decisions are not always flawless. For example, they tend to adopt unintended biases from the data they are trained on. Many believe that involving a human in the process, the so-called Human in the Loop, could be the solution to many automation issues. They could optimise the output of an automated system or oversee its decisions. But does human involvement automatically lead to better outcomes? And is it only the outcomes that matters? How do we ensure that human interventions genuinely add value? Who decides what in the automated process and on what basis? In the following article, we explain how we are exploring these questions in the research project Human in the Loop? at the Alexander von Humboldt Institute for Internet and Society.
Human-Machine Interactions: Where Errors Arise and Consequences Follow
Instances of consequential, inadequate human-machine interactions have recently become more frequent. For example, in the British Post Office scandal, a finance software incorrectly accused post office managers of embezzlement and faulty bookkeeping, leading to convictions of over 900 employees as well as payment demands. Another negative example was the Dutch childcare benefits scandal, where Dutch tax authorities used an AI system to identify potential welfare fraud cases, which, combined with human oversight, resulted in discriminatory outcomes. Over 20,000 parents were wrongly asked to repay significant amounts, plunging many into financial difficulties. These scenarios clearly demonstrate that many current automation processes are still highly error-prone. The reasons are manifold: for instance, humans tend to overly trust machine-made pre-decisions in some contexts, known as automation bias. Another issue can be the opacity of machine decision-making. Specifically, how can a human effectively intervene if they do not sufficiently understand the workings of the system they are monitoring – including the logic and reasoning behind its decision (or recommendation)? Moreover, this lack of clarity makes it difficult for those affected by erroneous automated decisions to legally challenge them, as they cannot prove they are unlawful (for example, due to discrimination). However, human biases can still affect human-machine interactions. If the training data of an AI system, for example, are not adequately prepared, biases inherent in those data can persist in the AI system, perpetuating learned discriminatory human decisions.
The European Union is trying to address some of these issues with the AI Act adopted in May. This marks the world’s first comprehensive attempt at AI regulation. The regulation specifically mandates that AI systems used for high-risk applications must be designed in a way they can be effectively supervised. This is especially true for areas where errors could have severe consequences. Thus, the Human in the Loop plays a crucial role as a hopeful figure in steering such human-machine interactions towards good decisions. We further clarify what ‘good’ entails later in the article.
Human Involvement: Who Takes on Which Role?
Firstly, who exactly is this Human in the Loop? For our research project, we define them as individuals actively participating in an automated process to enhance the system’s performance or monitor the quality of its decisions. Our definition incorporates technical descriptions, which primarily locate human involvement in the development stage of an AI system, such as data preparation or monitoring machine learning processes. It also considers regulatory perspectives, understanding the Human in the Loop mainly as a supervisor of an operational system, as described by the Bundesrat in its file 165/19. Therefore, examples of Humans in the Loop in our view include both, doctors using AI systems for initial X-ray assessments and human actors cleaning training data for such systems. However, how effective such human intervention can be remains not fully settled and depends on the specifics of each case of automation.
Decisions Under Scrutiny: Case Studies on AI-Supported Decision Processes
In our research project, we examine the interplay described between those Humans in the Loop and ‘machines’ in automated processes to better understand it. The goal is to generate new insights into how this interplay must be shaped to achieve good decisions. Through various case studies, we identify and gather the most relevant influencing factors affecting decision quality.
The initial case study focuses on the field of credit granting decisions. The use of AI systems offers efficiency gains but raises fundamental questions. For instance, does this process involve a Human in the Loop who reviews individual credit decisions? In a second case study, we delve into the realm of content moderation on digital platforms and social networks. Here, we analyse decision interactions between algorithms and humans, for example, aiming to enforce community rules and remove problematic content such as hate speech or misinformation.
Based on these findings, we develop concrete recommendations on how decision systems can be designed to facilitate successful human-machine interactions. Various factors influence the final decision, including how information is presented, personal values, legal liability issues, economic incentives, and the time available to make a decision. Each case study brings us closer to achieving overarching project goals. Firstly, we develop a comprehensive taxonomy – a practical overview of decision-relevant factors and characteristics. Secondly, we create specific action recommendations for the cases studied, contributing to improving collaboration between humans and machines in decision-making.
Efficiency and Ethics: Managing the Complexity of Our Research Questions
Examining the interplay between humans and machines in AI-supported decision processes presents several challenges. Particularly, detailed information on human involvement in areas such as credit granting is hard to access. Companies in this sector, for instance, seek to protect their internal processes and decision criteria to maintain competitive advantages and avoid the exploitation of potential weaknesses in their systems. Therefore, many of these processes remain undocumented practices or business secrets. In our research project, we have therefore actively engaged stakeholders willing to share their expertise. They assist us in understanding which actors (such as humans, AI systems, or companies) are involved in decision processes in the case studies of interest, how they collaborate, and which factors critically influence decisions. Simultaneously, we grapple intensely with the fundamental question of what criteria define a ‘good’ decision. Combined, this helps us assess how decisions in human-machine interactions should function factually, procedurally, and structurally. The subsequent question is much more complex: How do we measure the quality of those decisions? Assessing decision quality heavily depends on the perspective of the observer, as illustrated by an example from credit granting: Is the decision good for the individual, the bank, or society? The outcome can significantly differ depending on the viewpoint.
Conclusion: The Future of the Human in the Loop
In the future, automated decision-making processes will be established in many more industries, thus occupying more space in our daily lives. Therefore, it is crucial that we, as a society, understand their impacts and risks to ensure fair and transparent decision-making processes for all. We must ensure that capable individuals with specific qualifications receive sufficient and meaningful opportunities to influence automation processes. They must be empowered to accurately assess the quality of automated outputs and intervene as necessary. We will investigate the conditions under which this can be achieved in the coming years. Our research aims to facilitate the integration of algorithmic systems into human-guided decision processes in an ethically responsible and practically feasible manner.
This post represents the view of the author and does not necessarily represent the view of the institute itself. For more information about the topics of these articles and associated research projects, please contact info@hiig.de.
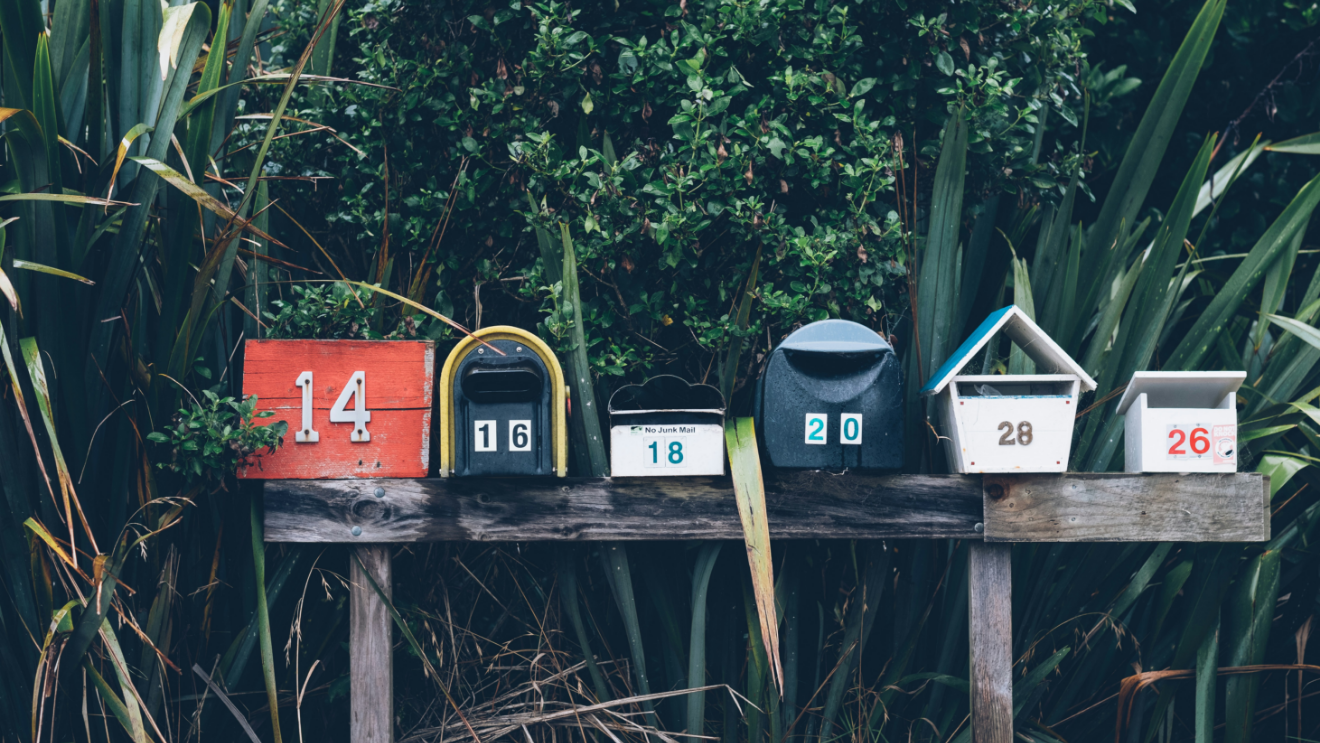
You will receive our latest blog articles once a month in a newsletter.
Artificial intelligence and society
Why access rights to platform data for researchers restrict, not promote, academic freedom
New German and EU digital laws grant researchers access rights to platform data, but narrow definitions of research risk undermining academic freedom.
Empowering workers with data
As workplaces become data-driven, can workers use people analytics to advocate for their rights? This article explores how data empowers workers and unions.
Two years after the takeover: Four key policy changes of X under Musk
This article outlines four key policy changes of X since Musk’s 2022 takeover, highlighting how the platform's approach to content moderation has evolved.