Making sense of our connected world
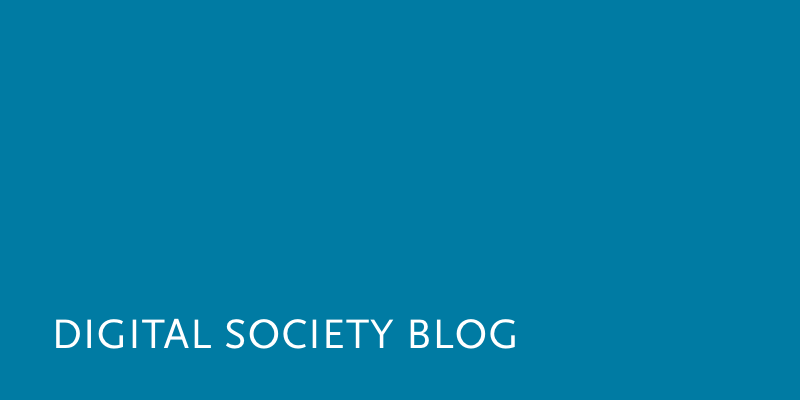
Data Sharing Angst – An Insight to an Ongoing Research on Data Sharing in Academia
From November 2013 to today, my colleague Sascha Friesike and me were conducting a systematic review with the intent to identify factors that influence a researcher’s data sharing behavior. The whole fun is part of my doctoral research. In accordance to what we consider Open Science, I want to use this blog post to give you a brief insight into our results, how we achieved them and my further intent to use them. I will discuss one finding that I find particularly interesting – the “Data Sharing Angst”.
I look forward to constructive feedback, creative ideas and weird questions – so please feel free to use the comment section or send me an Email.
Systematic Reviews in Empirical Research
Systematic reviews have proven their worth in evidence-based medicine. They are used to select and analyze research papers according to a pre-defined research question. One could say that it considers research literature as raw data. I will not go into a step-by-step description of a systematic review in the course of this blog post. However, for those who want to read up on it, I suggest this article.
In my opinion, a systematic review is also an elegant way to start an empirical investigation. It allows gaining an exhaustive overview of a research field, its leading scholars and prevailing discourses. It can thus serve as an analytical spadework for further inquiries (as in our case). Combined with a network analysis, one can identify schools of thought. With a content analysis one can bring order in fuzzy issues.
I could go on with my hymn of praise for the systematic review but let’s just say that I find it quite versatile, especially for starting a longer empirical quest. Today, it is used across disciplines. Dahlander and Gann’s review on innovation papers is a good example.
How we used the Systematic Review
We used a systematic review to identify factors that influence the data sharing behavior of researchers. Data sharing in research brings about many benefits, including the testability of study results or the application of old data for new questions. Boldly put, it has the potential to make research better and more efficient. It fosters scientific discovery. Still, it is not yet common rationale in research, as this study from 2011 shows.
Also for this reason, it is no surprise that data sharing in research is en vogue across all disciplines. Legal scholars discuss data ownership and rights of use, databank specialist meta data structures, coders data ontologies and social scientists action barriers. In our sample, we had 8 papers from ethnology and 9 from neuroscience. We therefore had to modify the systematic review a bit, so that it incorporates the different types of data and is best suited for further investigations. That is in our case a survey.
Different from most systematic reviews conducted in medicine, we applied an inductive content analysis in order to identify and structure data sharing factors in the literature. We decided for inductive coding, because we aimed at arriving at our own category system. We further conducted a Meta analysis, using the papers’ metadata. In the following, I will however relate to the results from the content analysis.
In the end, we ended up coding 101 papers from 7 literature databases (see below) from December 1, 2001 and December 1, 2013.
Database | Papers in sample |
Ebsco | Butler 2007; Chokshi et al. 2006; De Wolf et al. 2005 (also JSTOR, ProQuest); De Wolf et al. 2006 (also ProQuest); Feldman et al. 2012; Harding et al. 2011; Nelson 2009; Perrino et al. 2013; Pitt & Tang 2012; Sarathy & Muralidhar 2006; Sieber 1988; Stanley & Stanley 1988; Teeters et al. 2008; Xiaoqian et al. 2013 |
JSTOR | Axelsson & Schroeder 2009 (also ProQuest); Cooper 2007; Costello 2009; Duke 2006; Fulk et al. 2004; Guralnick & Constable 2010; Linkert et al. 2010; Ludman et al. 2010; Parr 2007; Resnik 2010; Rodgers & Nolte 2006; Sheather 2009; Whitlock et al. 2010; Zimmerman 2008 |
PLOS | Alsheikh-Ali et al. 2011; Chandramohan et al. 2008; Constable et al. 2010; Drew et al. 2013; Haendel et al. 2012; Masum et al. 2013; Milia et al. 2012; Molloy 2011; Noor et al. 2006; Piwowar 2011; Piwowar et al. 2007; Piwowar et al. 2008; Savage & Vickers 2009; Tenopir et al. 2011; Wallis et al. 2013; Wicherts et al. 2011 |
ProQuest | Acord & Harley 2013; Belmonte et al. 2007; Edwards et al. 2011; Elman et al. 2010; Foley et al. 2006; Kim & Shanton 2013; Nicholson & Bennett 2011; Piatek 2011; Rai & Eisenberg 2006; Reidpath & Allotey 2001; Tucker 2009 |
ScienceDirect | Anagnostou 2013; Brakewood & Poldrack 2013; Enke et al. 2011; Fisher & Fortman 2010; Karami et al. 2012; Par & Cummings 2008; Piwowar & Chapman 2009; Rohlfing & Poline 2011; Sayogo & Pardo 2012; Van Horn & Gazzaniga 2012; Wicherts & Bakker 2011 |
Springer | Albert 2012; Bezuidenhout 2013; Breeze et al. 2012; Fernandez et al. 2012; Freymann et al. 2012; Gardner et al. 2003; Jarnevich et al. 2007; Jones et al. 2012; Pearce & Smith 2011; Sansone & Rocca-Serra 2012; Teeters et al. 2008 |
Wiley | Borgman 2012; Daiglesh et al. 2012; Delson et al. 2007; Eschenfelder & Johnson 2008; Haddow 2010; Hayman et al. 2011; Huang et al. 2012; Kowalcyk & Shankar 2013; Levenson 2010; NIH 2002; NIH 2003; Ostelle & Beckmann 2009; Overbey 1999; Piwowar 2010; Reidpath & Allotey 2001; Rushby 2013; Samson 2008; Weber 2013; |
Misc. | Cragen et al. 2010 |
The result: A Framework for Sharing Research Data
After having coded the factors, we arrived at a category tree consisting of 6 main categories that already indicate a quite complex interaction system. I listed each main category together with a short explanation and some typical sharing barriers.
- Norms: Factors regarding the legal, ethical and policy norms
Barriers: Unclear data ownership and rights of use, Copyright issues, Personality rights of research participants - Individual Resources: Factors regarding the researcher’s investment and effort for sharing data.
Barriers: High curation effort, High learning effort - Disciplinary Practice: Factors regarding data-sharing practices in a discipline.
Barriers: Missing data standards, Missing data sharing culture - Research Agencies: Factors regarding crucial organizational players.
For instance: No career Benefits, Missing Datasharing Policies - Data Infrastructure: Factors regarding the technical infrastructure for data sharing.
For instance: Missing metadata standards, Usability of repositories, Security Issues - Returns: Factors regarding the return of investment for sharing research data.
For instance: Competitive Disadvantage, Commercial Misuse, Critique or Falsification, Flawed Interpretation Concerns
- Norms: Factors regarding the legal, ethical and policy norms
A qualitative content analysis allows no conclusion about the weighting of the single factors. Furthermore, we still need to tweak the categories a bit. Nevertheless, I find the category system itself quite useful to allocate sharing barriers – some of which are rather cognitive and can be boldly described as data sharing Angst. Lastly I will discuss those researchers’ fears as I believe they pose some interesting questions.
Data sharing Angst and how to overcome it
Data sharing in academia is good for everyone. It allows better and more research. For the single researcher, however, these advantages are not so apparent. The category Returns indicates that sharing research data is rather related to negative than positive individual outcomes.
For instance: Inhibiting returns include concerns about competitive disadvantage regarding other researchers, commercial misuse of data, the falsification of results and flawed data interpretation by others – all of which can be subsumed as Data sharing Angst. They exist, whether justified or not.
It is not surprising that a (voluntary) exchange system, in which the (perceived) adverse individual outcomes outweigh the (perhaps not apparent) individual benefits, does not work, as it could.
All of these concerns can be ascribed to a loss of control; the fear of what happens to my data once it is accessible for others. This brings up questions regarding the limits of openness, for instance:
- Would an embargo on research data diminish the fear of competitive disadvantage?
- Would a decleration of intent from the data recipient invalidate the fear of commercial misuse?
- Would a decentral storage of data (for example on the researcher’s own server) erase data security concerns?
- And finally, to what degree should researchers decide for themselves if they want to share their data or not?
I haven’t found intelligent answers to these questions yet. Also the data-sharing Angst is at best a good hypotheses. Nevertheless do I find these questions exciting and would be interested to read your opinion on that.
Limitations
The reservation must be made that our scope of inquiry (academic papers) and methodological design (focusing on the researcher) only portraits a limited perspective on a complex issue. The category system we derived would require further empirical testing. We are working on that.
This post is part of a weekly series of articles by doctoral canditates of the Alexander von Humboldt Institute for Internet and Society. It does not necessarily represent the view of the Institute itself. For more information about the topics of these articles and asssociated research projects, please contact presse@hiig.de.
This post represents the view of the author and does not necessarily represent the view of the institute itself. For more information about the topics of these articles and associated research projects, please contact info@hiig.de.
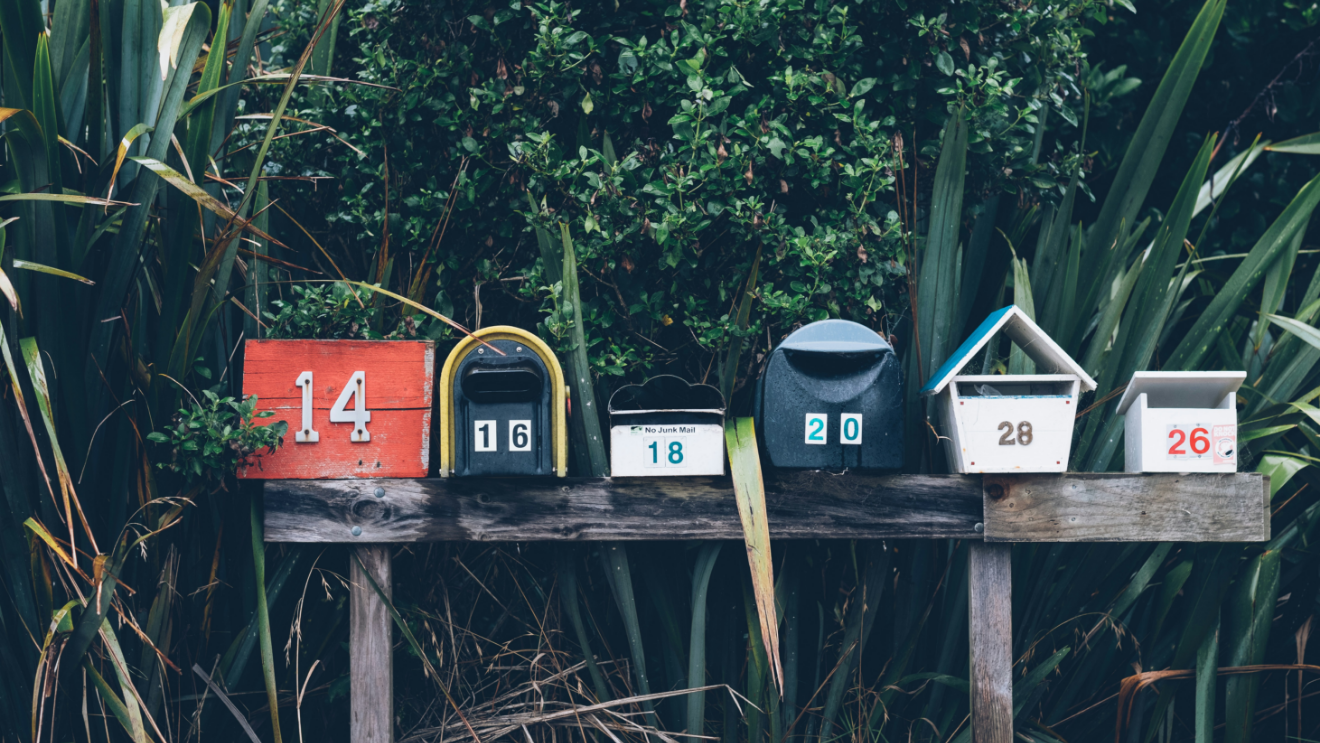
You will receive our latest blog articles once a month in a newsletter.
[wysija_form id="6"]
Research issues in focus
Do Community Notes have a party preference?
This article explores whether Community Notes effectively combat disinformation or mirror political biases, analysing distribution and rating patterns.
How People Analytics can affect the perception of fairness in the workplace
People Analytics in the workplace can improve decisions but may also heighten feelings of unfairness, impacting employee trust and workplace relationships.
What could German digital policy look like after the Bundestag election?
What are the digital policy positions of Germany's parties for the 2025 Bundestag election?