Making sense of our connected world
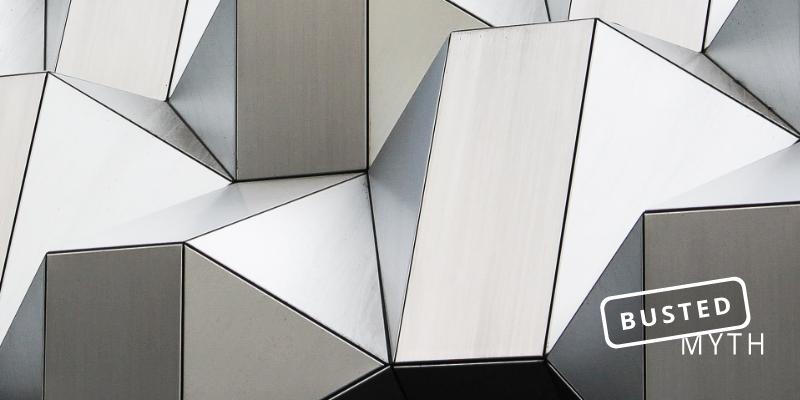
Myth: AI Models are abstract and do not need personal data
In supervised machine learning, models are based on abstractions from training data. The models themselves, while structurally influenced by the training data, do not contain the data themselves. It therefore seems reasonable to treat data they contain as (almost) anonymous. However, this is not true. Research has shown that deanonymization is possible under certain circumstances. Therefore, the models have to be considered as partially containing personal data and data protection law has to be taken into account when developing AI models to safeguard data subjects.
Myth
AI Models are abstract and do not need personal data.
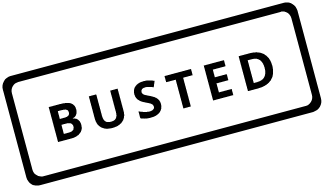
AI models are an abstraction which may or may not contain personal data. Data protection law needs to be taken into account.
Watch the talk
Material
Presentation Slides | |
KEY LITERATURE Shokri, R., Stronati, M., Song, C. & Shmatikov, V. (2016). Membership Inference Attacks Against Machine Learning Models. Al-Rubaie, M. & Chang, J. M. (2019). Privacy-Preserving Machine Learning: Threats and Solutions. EEE Security & Privacy, 17(2), 49-58. Liu, B., Ding, M., Shaham, S., Rahayu, W., Farokhi, F. & Lin, Z. (2021). When Machine Learning Meets Privacy: A Survey and Outlook. ACM Computing Surveys, 54(2), 1-36. |
About the author
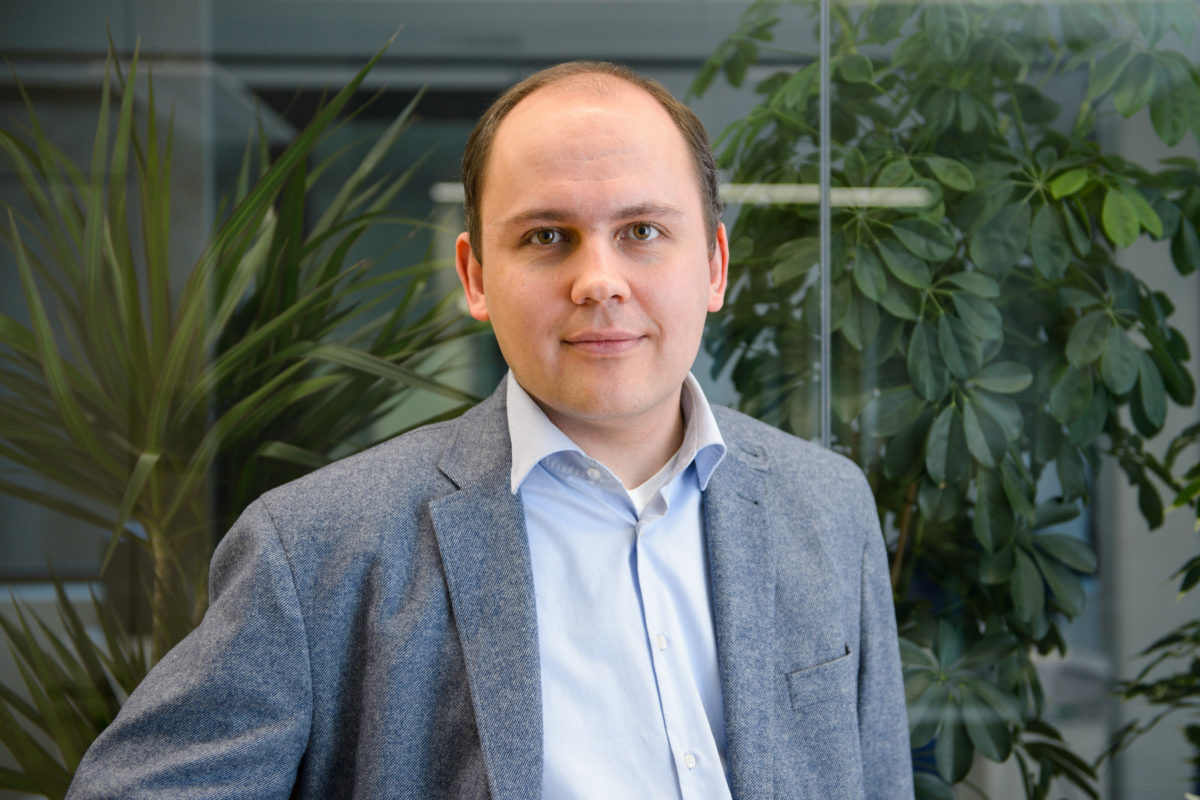
Christoph Sorge
Professor, Saarland University (Chair of Legal Informatics), Saarbrücken, Germany
Christoph Sorge received his PhD in computer science from Karlsruhe Institute of Technology. He then joined the NEC Laboratories Europe, Network Research Division, as a research scientist. From 2010, Christoph was an assistant professor (“Juniorprofessor”) for Network Security at the University of Paderborn. He joined Saarland University in 2014, and is now a full professor of Legal Informatics at that university. While his primary affiliation is with the Faculty of Law, he is also a co-opted professor of computer science. He is an associated member of the CISPA – Helmholtz Center for Information Security, a senior fellow of the German Research Institute for Public Administration, and a board member of the German Association for Computing in the Judiciary. His research area is the intersection of computer science and law, with a focus on data protection.
This post represents the view of the author and does not necessarily represent the view of the institute itself. For more information about the topics of these articles and associated research projects, please contact info@hiig.de.
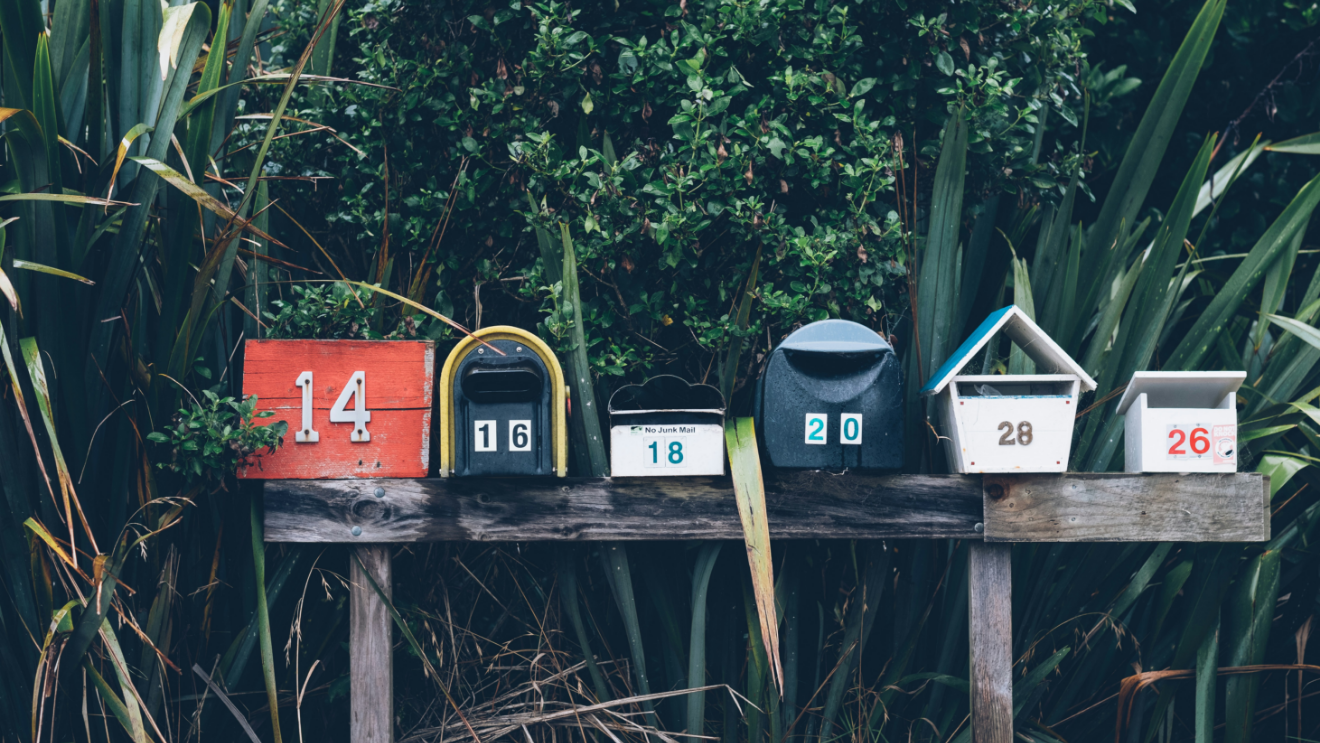
You will receive our latest blog articles once a month in a newsletter.
Artificial intelligence and society
Escaping the digitalisation backlog: data governance puts cities and municipalities in the digital fast lane
The Data Governance Guide empowers cities to develop data-driven services that serve citizens effectively.
Online echoes: the Tagesschau in Einfacher Sprache
How is the Tagesschau in Einfacher Sprache perceived? This analysis of Reddit comments reveals how the new simplified format news is discussed online.
Opportunities to combat loneliness: How care facilities are connecting neighborhoods
Can digital tools help combat loneliness in old age? Care facilities are rethinking their role as inclusive, connected places in the community.