Phillipp Schoppmann
Phillipp Schoppmann studied Computer Science and Informatics at Humboldt University of Berlin and the University of Edinburgh. Since October 2016 he has been a PhD student and a research assistant at the computer engineering group of HU Berlin, conducting research on privacy, security, cryptography, and multi-party computation. During his PhD studies, he spent multiple months abroad on research stays at the Alan Turing Institute in London and at Google in New York. Since June 2019, Phillipp has been an associated researcher at HIIG, working on “Secure multiparty computation for machine learning and healthcare applications” as part of the research program “Data, actors, infrastructures”.
Journal articles and conference proceedings
Schoppmann, P., Vogelsang, L., Gascón, A., & Balle, B. (2020). Secure and Scalable Document Similarity on Distributed Databases: Differential Privacy to the Rescue. Proceedings on Privacy Enhancing Technologies, 2020(2), 209–229. DOI: 10.2478/popets-2020-0024 Publication details
Vogelsang, L., Lehne, M., Schoppmann, P., Prasser, F., Thun, S., Scheuermann, B., & Schepers, J. (2020). A Secure Multi-Party Computation Protocol for Time-To-Event Analyses. IOS Press Ebook Series: Studies in Health Technology and Informatics, 270: Digital Personalized Health and Medicine, 8–12. DOI: 10.3233/SHTI200112 Publication details
Schoppmann, P., Gascón, A., Reichert, L. , and Raykova, M. (2019). Distributed Vector-OLE: Improved Constructions and Implementation. CCS '19: Proceedings of the 2019 ACM SIGSAC Conference on Computer and Communications Security, 1055–1072. DOI: 10.1145/3319535.3363228 Publication details
Schoppmann, P., Gascón, A., Raykova, M., and Pinkas, B. (2019). Make Some ROOM for the Zeros: Data Sparsity in Secure Distributed Machine Learning. CCS '19: Proceedings of the 2019 ACM SIGSAC Conference on Computer and Communications Security, 1335–1350. DOI: 10.1145/3319535.3339816 Publication details
Gascón, A. , Schoppmann, P., Balle, B. , Raykova, M., Doerner, J., Zahur, S. , and Evans, D. (2017). Privacy Preserving Distributed Linear Regression on High-Dimensional Data. Proceedings on Privacy Enhancing Technologies (PETS '17), 2017, 2017(4), 345-364. DOI: 10.1515/popets-2017-0053 Publication details
Lectures and presentations
Make Some ROOM for the Zeros - Data Sparsity in Secure Distributed Machine LearningTheory and Practice of Multi-Party Computation Workshop (Session: Theory and Practice of Multi-Party Computation Workshop). Bar-Ilan University. Bar-Ilan University, Tel Aviv, Israel: 15.11.2019 Further information
Phillipp Schoppmann
Make Some ROOM for the Zeros - Data Sparsity in Secure Distributed Machine LearningLondon Crypto Day 2019 (Session: London Crypto Day 2019). Google London, London, United Kingdom: 11.10.2019 Further information
Phillipp Schoppmann
Make Some ROOM for the Zeros - Data Sparsity in Secure Distributed Machine LearningOblivious Data Structures and Algorithms Workshop (Session: Oblivious Data Structures and Algorithms Workshop). ODSA: Oblivious Data Structures and Algorithms. University Residential Center l, Bertinoro, Italy: 17.07.2019 Further information
Phillipp Schoppmann
Organisation of events
CCS 2019 Workshop: PRIVACY PRESERVING MACHINE LEARNINGCCS 2019 Workshop: PRIVACY PRESERVING MACHINE LEARNING. 15.11.2019. London, London, United Kingdom. Co-Organised by: Borja Balle, Adrià Gascón, Olya Ohrimenko, Mariana Raykova, Carmela Troncoso (International) Further information
Phillipp Schoppmann
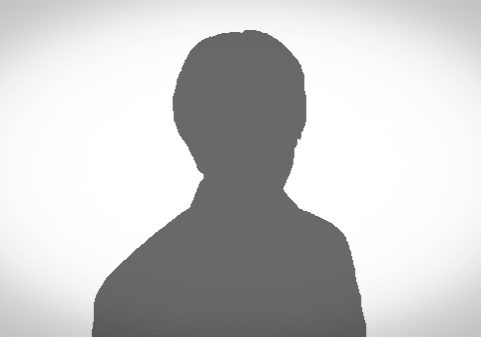
Position
Former Associated Researcher: Data, actors, infrastructures