KS+: Predicting Workflow Task Memory Usage Over Time
Author: | Bader, J., Lösser, A., Scheuermann, B., & Kao, O. |
Published in: | Computer Communications 225, 1-7 |
Year: | 2024 |
Type: | Academic articles |
DOI: | https://doi.org/10.1109/e-Science62913.2024.10678686 |
Scientific workflow management systems enable the reproducible execution of data analysis pipelines on cluster infrastructures managed by resource managers such as Kubernetes, Slurm, or HTCondor. These resource managers require resource estimates for each workflow task to be executed on one of the cluster nodes. However, task resource consumption varies significantly between different tasks and for the same task with different inputs. Furthermore, resource consumption also fluctuates during a task's execution. As a result, manually configuring static memory allocations is error-prone, often leading users to overestimate memory usage to avoid costly failures from under-provisioning, which results in significant memory wastage. We propose KS+, a method that predicts a task's memory consumption over time depending on its inputs. For this, KS+ dynamically segments the task execution and predicts the memory required for each segment. Our experimental evaluation shows an average reduction in memory wastage of 38% compared to the best-performing state-of-the-art baseline for two real-world workflows from the popular nf-core repository.
Visit publication |
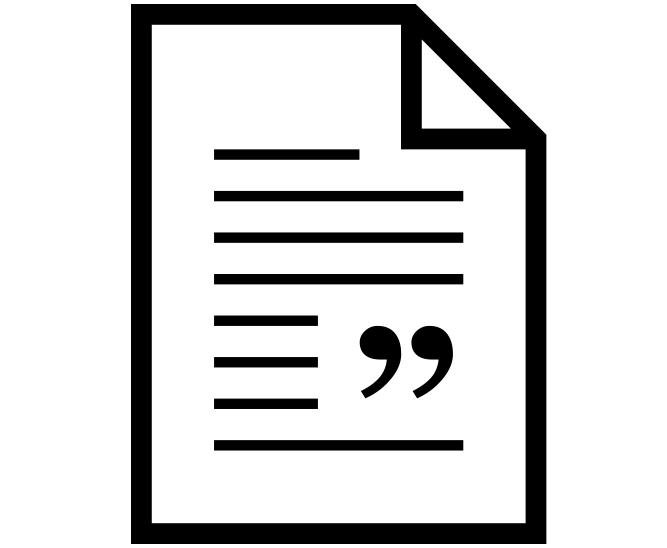
Connected HIIG researchers
Björn Scheuermann, Prof. Dr.
- Open Access