Wikipedia: A Challenger’s Best Friend? Utilising Information-seeking Behavior patterns to Predict US Congressional Elections
Author: | Salem, H., & Stephany, F. |
Published in: | |
Year: | 2020 |
Type: | Working paper |
Election prediction has long been an evergreen in political science literature. Traditionally, such efforts included polling aggregates, economic indicators, partisan affiliation, and campaign effects to predict aggregate voting outcomes. With increasing secondary usage of online-generated data in social science, researchers have begun to consult metadata from widely used web-based platforms such as Facebook, Twitter, Google Trends and Wikipedia to calibrate forecasting models. Web-based platforms offer the means for voters to retrieve detailed campaign-related information, and for researchers to study the popularity of campaigns and public sentiment surrounding them. However, past contributions have often overlooked the interaction between conventional election variables and information-seeking behaviour patterns. In this work, we aim to unify traditional and novel methodology by considering how information retrieval differs between incumbent and challenger campaigns, as well as the effect of perceived candidate viability and media coverage on Wikipedia pageviews predictive ability. In order to test our hypotheses, we use election data from United States Congressional (Senate and House) elections between 2016 and 2018. We demonstrate that Wikipedia data, as a proxy for information-seeking behaviour patterns, is particularly useful for predicting the success of well-funded challengers who are relatively less covered in the media. In general, our findings underline the importance of a mixed-data approach to predictive analytics in computational social science.
Visit publication |
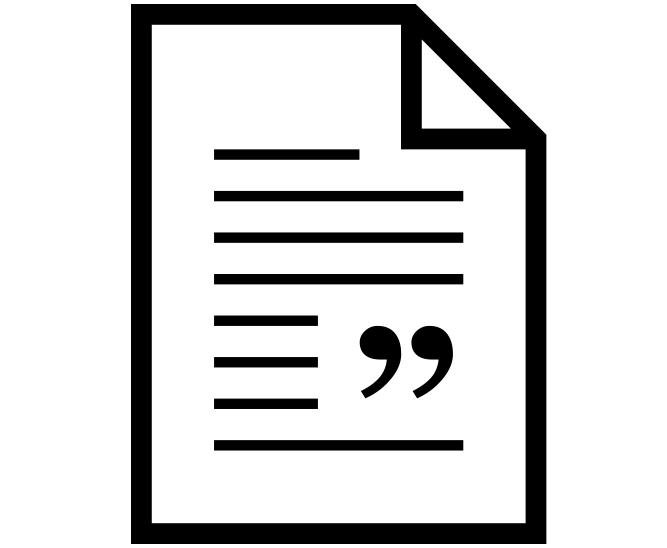
Connected HIIG researchers
Fabian Stephany, Dr.
- Open Access