Making sense of our connected world
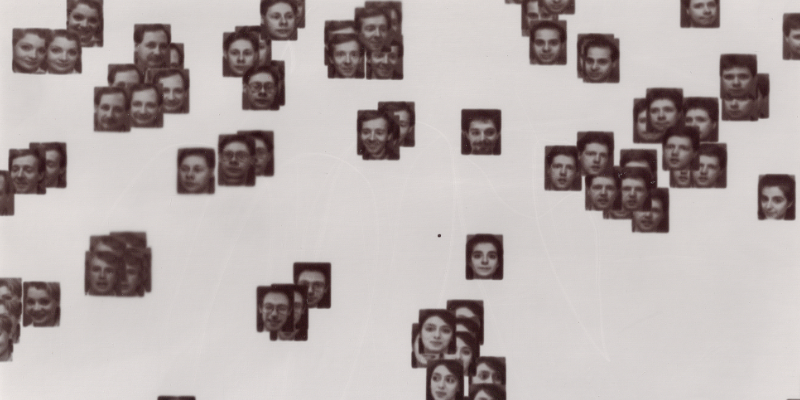
Shaping AI in the interests of employees
AI offers opportunities and risks for employees. But what can managers and works councils do to enable potential positive effects and avoid negative effects? This article describes possible design approaches based on the forthcoming handbook “AI in the Context of Knowledge Work: Fields of Action and Approaches for an Employee-Oriented Design“.
Artificial Intelligence is being increasingly used – also in the context of knowledge work
At the beginning of our research project Artificial Intelligence and Knowledge Work (KIWI) in 2019, artificial intelligence (AI) in everyday work still sounded like the distant future; today, it is already a reality in many companies – including in the context of knowledge work. In fact, the number of companies using AI has nearly doubled since 2019 (Rammer, 2022). As part of the project, we began by investigating whether – and if so, how – AI has been adopted in the context of knowledge work in Germany to date. In the process, we got to know a variety of applications: from AI systems that are used to transcribe and index texts, to those that identify hate speech in comments, up to the ones that consultants use to organize the accounts of their customers. The range is conceivably large. A selection of these applications can be found in our public AI-Case-Collection.
AI in the workplace
In the process of the KIWI project, it has become clear that there are two main scenarios for the use of AI in the context of knowledge work: augmentation and automation (Raisch & Krakowski, 2021; von Richthofen et al., 2021).
Augmentation of work
Augmentation involves that people work closely together with machines to perform a task. A good example from our case studies concerns the organization of knowledge in libraries. In the past, librarians have intellectually indexed new publications. This means that certain keywords are assigned to each new publication to make it easier for users to find them. Today, AI is increasingly used to either fully automate this process or at least suggest keywords to librarians.
Automation of work
In the case of automation, machines take over tasks that were previously performed by humans. A common example of automation is the use of chatbots and voicebots in customer service. In general, this involves the automation of individual and clearly definable tasks, such as the authentication of customers. In the organizations that we studied as part of the KIWI project, we observed that AI was used in most cases to automate rather than augment tasks. But there are also hybrid forms, in which the boundaries between automation and augmentation blur (von Richthofen et al., 2022).
AI holds opportunities and risks for employees
For many years, AI was presented as either a savior or a danger for employees. However, at the latest since the publication of the report Artificial Intelligence – Social Responsibility and Economic, Social and Ecological Potentials, it has become clear that AI offers both opportunities and risks for employees. The implications of the adoption of AI depend on the social and organizational conditions as well as its concrete design.
The goal is to enable the positive effects of AI use for employees (e.g., relieving them of exhausting and/or dangerous tasks) and to avoid the negative effects of AI use (e.g., surveillance of employees and/or the elimination of jobs). During the process of the project, we conducted case studies and interviews with representatives of numerous organizations that are already using or currently implementing AI. Based on our findings and workshops with representatives from management and work councils, we have identified fields of action that can support managers and work representatives to introduce AI in an employee-oriented manner, i.e. in the interests of and with consideration for the needs of employees.
Fields of action for managers
There are a number of approaches for management to introduce AI in the interests of employees, which we summarize in three fields of action: Orienting, Enabling, and Involving. Our insights are based on eight case studies and 50 related interviews with users, developers, managers and project leaders.
Orienting
Orienting refers to a field of action that aims at informing employees about AI as a technology. This includes illustrating its current, as well as its potential application possibilities within the respective organization and placing it in a larger context of meaning. Orientation with regard to AI is important, because the term AI is controversial even among experts (Liu, 2021) and because AI’s public perception tends to be shaped by myths (Huysman, 2020). To provide orientation to employees, managers can use three approaches, which we refer to as agreeing, looking ahead, and exemplifying.
Enabling
Enabling involves practices that aim to allow organizations, as well as their teams and employees to develop, implement, use, and maintain AI systems. Thus, to enable employees is a key field of action for employee-centered design, as AI deployment is associated with the emergence of new tasks and roles that in many cases require the acquisition of new skills (von Richthofen et al., 2022). Three approaches are suitable to qualify employees: the in-house development of AI applications, interorganizational cooperation, and training offerings.
Participating
In the organizational context, participation encompasses general practices and processes to directly involve employees in the design of the workplace, work structures and work processes (Becker & Brinkmann, 2017). In the context of AI introduction, early and comprehensive participation of workers and their representatives is desirable. In particular, users of the technology have essential domain knowledge for the successful development and deployment of AI applications (von Richthofen et al., 2022). Employees should therefore already be involved in the identification of use cases and their domain knowledge should be effectively integrated into the development process.
Fields of action for worker representation
An early and comprehensive involvement of works councils can contribute substantially to the success of AI implementations (Klengel & Wenckebach 2021). Works councils have deep knowledge of the workplace and needs of employees. Thus, they can evaluate the feasibility of AI projects realistically and increase acceptance of new systems among the workforce. At the same time, the distinct characteristics of AI systems also pose challenges to work representatives. Coping approaches by and for employee representatives can be summarized in three fields of action. These findings are based on 25 interviews and a workshop with works council members, trade unionists and former works council members who act as consultants for AI co-determination.
Understanding
Activities in the field of action Understanding describe the development and management of AI knowledge within the works council. The functionalities of AI systems are complex and often difficult to understand, not only from the perspective of works councils. At the same time, co-determination of AI at eye level requires that employee representatives have a basic knowledge of AI. In the handbook, we therefore identify three approaches that have proven helpful for works councils to build up and manage this knowledge: Training in the form of needs-based classes, networking with external and internal experts, and disseminating relevant information on current internal AI projects.
Systematizing
In the field of action Systematizing we describe approaches of using checklists to facilitate processes in the context of AI implementation. The need for this emerges due to a lack of routines, experiences and suitable structures for dealing with AI among the majority of works councils. This often already starts with the lack of a practical definition of AI. In the handbook, we therefore identify AI information sheets and traffic light systems as two approaches that works council members describe as useful for systematization. AI information sheets present key facts of the organization’s AI projects in a standardized format. Traffic light systems make it possible to classify and categorize AI systems using central criteria.
Flexibilizing
In the Flexibilize field of action, we describe ways to design deliberately open processes in the regulation of AI systems. Since the systems are constantly evolving, they can develop dynamics that are difficult to predict from the perspective of works councils. At the same time, AI systems can have far-reaching consequences for employees, such as on their autonomy or the quality of activities they perform. Therefore, AI systems should be tested in advance as well as possible and regulated with foresight. According to employee representatives, pilot projects and process-oriented company agreements are suitable for dealing with the changeability of AI systems in negotiations. Pilot projects involve the testing and temporary use of AI systems within a small and clearly defined scope. Process-oriented company agreements focus on regulating the processes for using AI systems instead of technical details.
Outlook
Even though organizations are increasingly implementing AI in their work processes, the adoption of AI in Germany is overall still in its beginnings. To date, only around 10 percent of companies in Germany are using AI (Rammer, 2022). This offers the opportunity to actively shape AI in the interests of employees. The rising adoption rates of AI in companies suggest that its design will increasingly shape the future of work. Even though we have observed mostly positive effects in our studies so far, such as employees being relieved of tedious and repetitive tasks, this does not mean that negative effects cannot occur. For example, leading researchers argue that many of the effects of the use of AI will materialise only in years. Thus, there is a growing need to systematically develop, test, and deploy approaches to design AI in an employee-centric way.
References
Becker, K., & Brinkmann, U. (2017). Partizipation. In H. Hirsch-Kreinsen & H. Minssen (Eds.), Lexikon der Arbeits- und Industriesoziologie (pp. 254–258). Nomos.
Huysman, M. (2020). Information systems research on artificial intelligence and work: A commentary on “Robo-Apocalypse canceled? Reframing the automation and future of work debate”. Journal of Information Technology, 35(4), 307–309. https://doi.org/10.1177/0268396220926511
Klengel, E., & Wenckebach, J. (2021). Artificial intelligence, work, power imbalance and democracy – why co-determination is essential. Italian Labour Law E-Journal, 2(14), 157-171. https://doi.org/10/gn7kcv
Liu, Z. (2021). Sociological perspectives on artificial intelligence: A typological reading. Sociology Compass, 15(3), e12851. https://doi.org/10.1111/soc4.12851
Raisch, S., & Krakowski, S. (2021). Artificial Intelligence and Management: The Automation–Augmentation Paradox. Academy of Management Review, 46(1), 192–210. https://doi.org/10.5465/amr.2018.0072
Rammer, C. (2022). Kompetenzen und Kooperationen zu Künstlicher Intelligenz: Ergebnisse einer Befragung von KI-aktiven Unternehmen in Deutschland. Bundesministerium für Wirtschaft und Klimaschutz. https://www.de.digital/DIGITAL/Redaktion/DE/Digitalisierungsindex/ Publikationen/publikation-download-ki-kompetenzen.html
von Richthofen, G., Gümüsay, A. A., & Send, H. (2021). Künstliche Intelligenz und die Zukunft von Arbeit. In R. Altenburger & R. Schmidpeter (Eds.), CSR und Künstliche Intelligenz (pp. 353-366). Springer Berlin Heidelberg. https://doi.org/10.1007/978-3-662-63223-9_19
von Richthofen, G., Ogolla, S., & Send, H. (2022). Adopting AI in the Context of Knowledge Work: Empirical Insights from German Organizations. Information, 13(4), 199. https://www.mdpi.com/2078-2489/13/4/199
This post represents the view of the author and does not necessarily represent the view of the institute itself. For more information about the topics of these articles and associated research projects, please contact info@hiig.de.
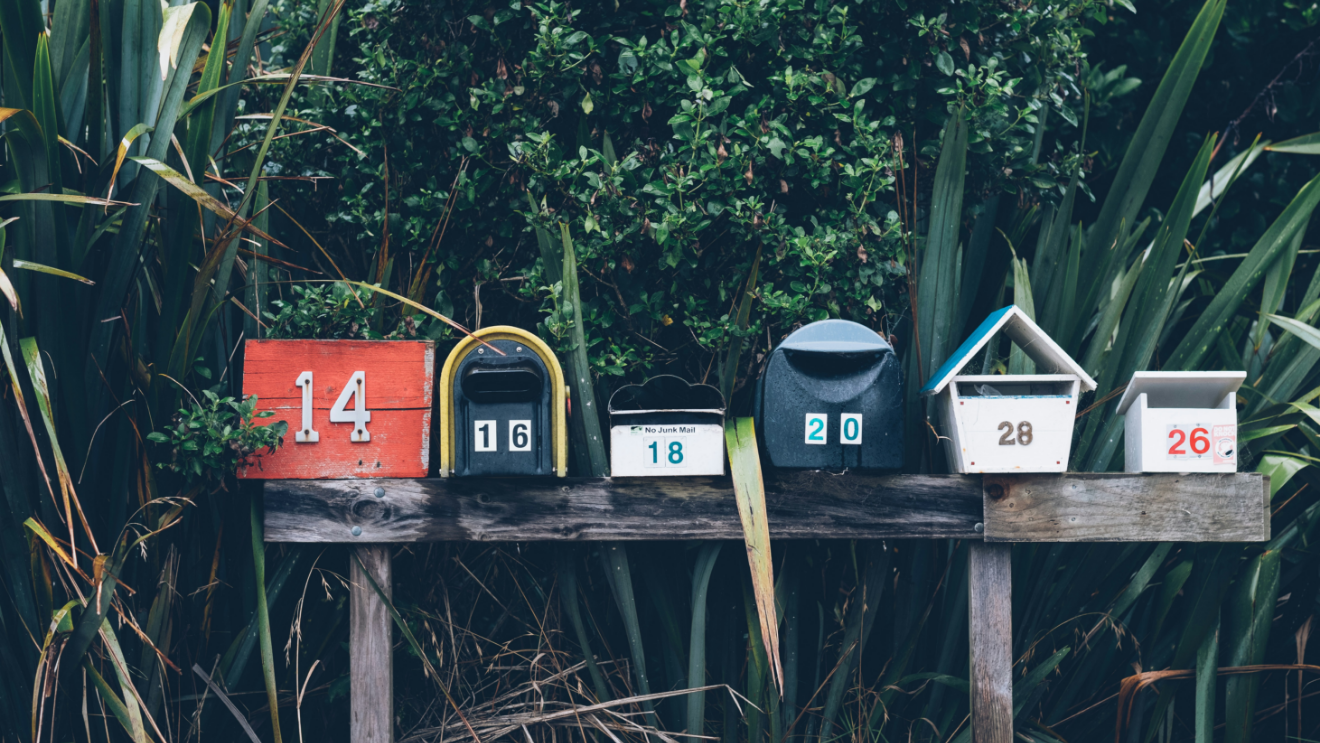
You will receive our latest blog articles once a month in a newsletter.
Digital future of the workplace
AI at the microphone: The voice of the future?
From synthesising voices and generating entire episodes, AI is transforming digital audio. Explore the opportunities and challenges of AI at the microphone.
Do Community Notes have a party preference?
This article explores whether Community Notes effectively combat disinformation or mirror political biases, analysing distribution and rating patterns.
How People Analytics can affect the perception of fairness in the workplace
People Analytics in the workplace can improve decisions but may also heighten feelings of unfairness, impacting employee trust and workplace relationships.