Making sense of our connected world
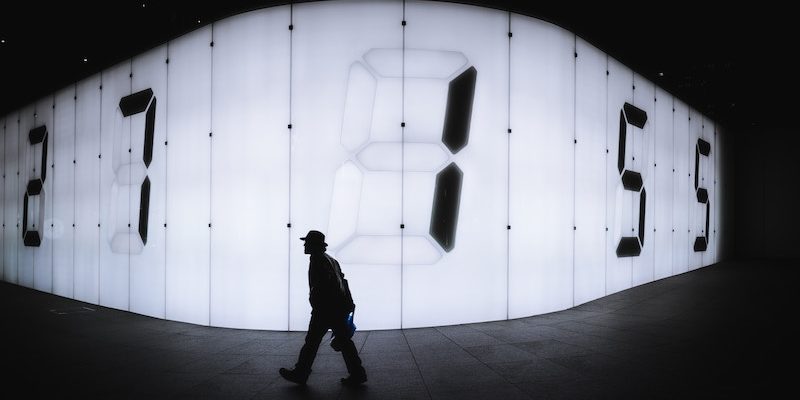
Status Work in the Data Society: Übercapital and Hyper-individualization
Whether it is childcare, credit or consumer goods: ratings, scores and the digital footprint produce new social classifications. This increasing quantification of the social is the reason for new forms of status gains or losses. In our lecture series “Making Sense of the Digital Society”, Marion Fourcade grasped these tendencies with the term “übercapital”. Steffen Mau weighs in with her theoretical thoughts and depicts how this leads to hyper-individualization and a new regime of inequality.
Anyone looking for a babysitter on the relevant websites in the USA is immediately confronted with the critical question of trustworthiness and asked to inform oneself about it. Entrusting your child to a stranger often leaves you with a bad feeling. Up until this point, references of former clients or advice from other parents has helped. Now, however, there is another option that is only a click away: The mylife.com website offers a reputation score for all adult Americans, which can also be used to test babysitters.
In the online description, it says: “Reputation is more important than credit. Only MyLife provides Reputation Scores based on public information gathered from government, social, and other sources, plus personal reviews written by others.” To find out someone else’s reputation score, just enter their name and zip code and you will find what you are looking for. The national criminal records, the public Internet and the Darknet as well as social media and its personal profiles accessible are evaluated.
In short, it concerns all personal data that can be evaluated via accessible databases. The customer receives a comprehensive background report with each inquiry, which lists all sub-areas. For a quick and comparative look there is a rating value of 0-5, including a speedometer display from red to green. The users are enthusiastic! Luisa: “You’d be crazy to rent someone an apartment without looking at the background report first – it saves me tons of trouble.”; Ricardo: “I declined a job offer after I did a background check on the guy who was going to be my supervisor.”; Lerrie “I felt a lot better about my daughter’s new boyfriend after I ran a background report.”
The reputation rating for babysitters represents a general trend: the quantification of the social. The compiling, accumulating, assembling and linking of personal data from very different sources, which becomes possible in the course of digitization, makes it possible to comprehensively track and evaluate people. Leisure time, consumer habits, health and income status, friendship networks, living contexts, information on the work environment and the employer – all this can be collected in a single data set, which can then be used again to promote the “pervasive capitalization of the lifeworld” (Wolfgang Streeck).
In her lecture “Social Order in the Digital Society”, Marion Fourcade builds on Bourdieu’s ideas, speaking of “übercapital” as a form of capital based on data that becomes visible in this type of data-based classification. Digital status data can become effective as symbolic capital and promise considerable social or material returns on reputation, which is why people are also constantly striving for better data. For babysitters, this means that they will only get hired if the score is good. One can use one’s reputation to achieve better positions, just as a bad reputation or a dubious image can bring about considerable disadvantages in housing, partnership, credit, employment or other markets. Symbolic capital in the form of status data can thus be converted into material benefits or useful contacts, because others use this information.
It is ultimately the “display of symbolic capital” that ensures that “capital comes to capital,” according to Pierre Bourdieu. In the case of scores, the chances of preferential treatment are considerable because direct comparisons of the symbolic account balance can be made. It often takes just one click to find out where someone stands. If scores are understood as symbolic capital expressed in the language of numbers, then it becomes clear that the symbolic in this abstract and generalized form can now be communicated and used much more comprehensively than the traditional, often locally limited or sectoral “good reputation”.
But what does this development mean for traditional forms of classification, such as classification into classes? Are we facing a “digital class society” today, which would mean that new collective categories are emerging? This is doubtful, because the data does only partly address collectives as such, but primarily individuals. The increase in data means that it is possible to classify ever more precisely – the digitization of society leads to a “difference revolution” (Christoph Kucklick). Comprehensive collective categories are dissolved in favor of finely chiselled differences. One can imagine this as a zoom that drives into a group photo and is able to resolve individual persons more and more precisely. Instead of fuzzy, large-grained images, one can now see all the details with great sharpness.
Against this background, the advancing digitization results in hyper-individualization, which is based on an infinite number of endlessly combinable observations of differences. Quantifying the social also means the possibility to divide the social. This kind of individualization does not come along as liberation or emancipation, but as the exploration of the individual through statistical identification. This type of individualization is a process of singularizing, identifying, locating and distinguishing people within a larger mass.
However, this data-driven hyper-individualization is ambivalent, because it requires a collective reference. Each performance data of self-monitoring, each health score or each rating cannot exist without standardization and the connection to collective data. The actuarial assessment of whether someone is driving well or badly depends on how all other people in the data pool drive the steering wheel. The babysitter’s reputation is also evident, especially in comparison to other babysitters who offer their services. Social comparisons, norms, risk calculations, purchasing power assessments – they all recognize the individual (or other valuation objects) only in relation to larger entities and on the basis of predefined valuation dimensions. The individual arises from the collective, in other words as observation of difference.
This is accompanied by a shift in the regime of social inequality away from class conflict to individual competition. (The competitors can of course also be universities, hospitals, professions in their service roles or states, depending on which allocation unit is in focus.) The conflict is about the direct confrontation between the parties involved, the struggle with each other, in the competition with regard to specific performance goals. Rankings, health scores, fitness points, performance indicators, ratings or like-clicks on social media strengthen the comparative dispositive from which competition emerges directly.
In the data-based competitive society, one struggles individually for scores, places or performance advantages, and no longer collectively for power or distributive justice as it happened in the society of class conflict. The struggle for power, participation and redistribution has thus turned into a game of overbidding and individual optimization that undermines the collectivization capacity of interests. The quantification of the social has the potential to create a new regime of inequality, one in which we are constantly rated and compared with others, in which our status data are used by others to assess and address us, and in which we must constantly strive to shine with good data.
Steffen Mau is Professor of Macrosociology at the Humboldt-Universität zu Berlin and author of the book „Das metrische Wir. Über die Quantifizierung des Sozialen“ (Suhrkamp 2017).
This post represents the view of the author and does not necessarily represent the view of the institute itself. For more information about the topics of these articles and associated research projects, please contact info@hiig.de.
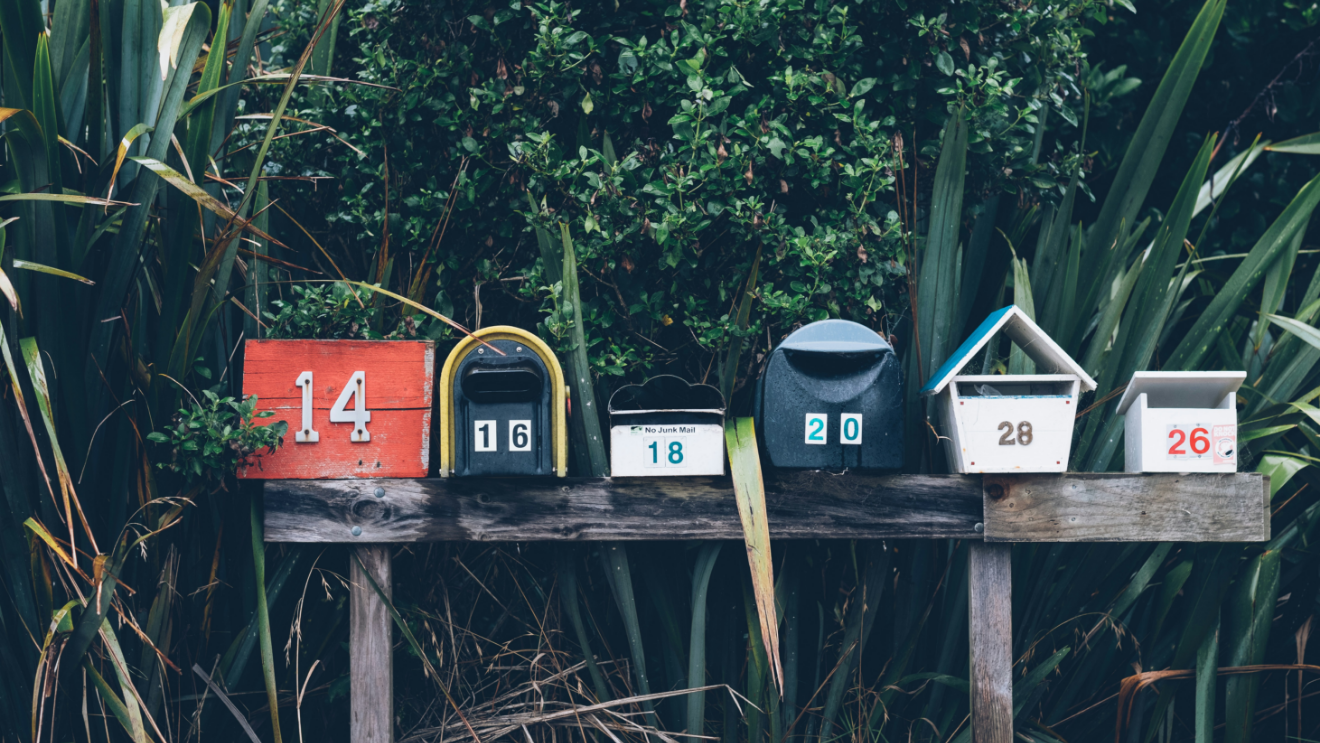
You will receive our latest blog articles once a month in a newsletter.
[wysija_form id="6"]
Platform governance
Do Community Notes have a party preference?
This article explores whether Community Notes effectively combat disinformation or mirror political biases, analysing distribution and rating patterns.
How People Analytics can affect the perception of fairness in the workplace
People Analytics in the workplace can improve decisions but may also heighten feelings of unfairness, impacting employee trust and workplace relationships.
What could German digital policy look like after the Bundestag election?
What are the digital policy positions of Germany's parties for the 2025 Bundestag election?