Making sense of our connected world
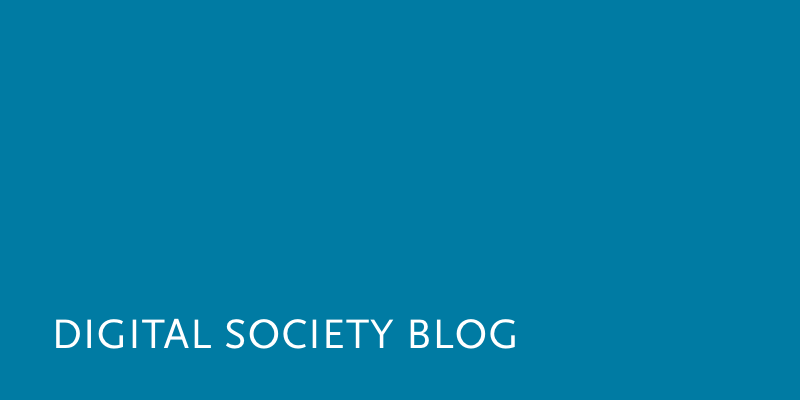
The logic of cascades
What factors influence the spread of information online? Together with my colleague Isabella Peters from the University of Kiel, I have been thinking about this question in the context of a book chapter that we are currently working on. We looked at several recent studies on information diffusion, and I want to take the opportunity to summarize and comment on the findings of those studies here.
Current news, political messages, and memes are shared every single moment on the Internet, particularly through social media. Messages can spread with incredible speed and have considerable public impact (think disasters, uprisings, or other breaking news), affecting us both as citizens and increasingly also making information diffusion an important object of information scholarship. Social media enable the rapid diffusion of news, while also being subject to manipulation (for example, through bots) and hacking (for example, when sensitive accounts are hijacked). Platform providers are increasingly under pressure to react to government interventions that seek to limit the spread of information through social media platforms, as was recently the case in Turkey.
Why do certain pieces of information spread much more widely than others, and what factors play a role in information diffusion? Scientists from different fields now study such processes under labels such as web science and computational social science. They analyze vast amounts of data, for example with the goal of determining the most salient factors which contribute to the spread of a particular piece of information. Increasingly it is possible to track such processes in real time on platforms such as Twitter, Facebook or Google Plus, assuming one has access to the data. This allows the description of an ad campaign with the same methodology as the success of a political campaign or the popularity of cat content (though it is best not to confuse sharing with long-term engagement).
In a recently published conference paper, the authors describe how informational cascades unfold using data on how images are shared on Facebook (Cheng et al, 2014). Cascades are sudden bursts of activity in which a piece of content suddenly becomes very popular. People often talk of Internet content being ‘viral’, but genuine cascades are quite rare and notoriously hard to predict. While the authors of the study stress that making definite predictions is still difficult, they are able to predict the size of a cascade with 80% accuracy once the respective piece of content has been shared five times. Other external factors are also relevant. For example, the popularity of the sharer is more important than the content as such. Further factors that make a difference and are unrelated to the content itself are the depth and breadth of the cascade, the language of the original piece of content, and the type of information shared (e.g. religious or political content). The most important single factor, however, is time. The speed of the initial five reshares allows a relatively precise estimation of the ultimate size of the cascade.
What applies to one platform may not apply to another. Four years ago Korean researchers examined Twitter to determine whether it behaved more like a social network in the sociological sense, or more like broadcast media (Kwak et al, 2010). They found that Twitter had an extreme concentration of attention on a small number of actors and low reciprocity, making it more similar to a mass medium than a social network. The structural differences between both platforms – reciprocal relationships in Facebook vs. non-reciprocal relationships in Twitter – means that the visibility of individual users is comparatively greater in Twitter than in Facebook, in addition to much of the content shared through the former coming directly from mass media sources. The differences in the design of both platforms therefore significantly impact information diffusion in them.
One aspect that is particularly interesting to me in this context is the degree of cultural and linguistic variation that we find in social media. These factors both play an important role and have hardly been studied to date. In another paper on Twitter, the authors compare the percentage of tweets that contain a URL or hashtag, the number of @-messages and mentions, and the number of retweets, and relate these figures to the language of the tweet (Hong et al, 2011). They find that tweets in German are three times as likely to contain URLs than tweets in Japanese or Portuguese. Hashtags are apparently also much more popular among German-speaking users than among users in other countries – perhaps an indicator for a local usage of Twitter more concerned with spreading information than with communicating with peers, which plays a very active role elsewhere. But these figures reflects an ephemeral picture that can change quite rapidly as platforms mature. In addition to shifting user behavior, web science studies also have to contend with issues of reproducibility, which can be highly problematic when data access is in practice limited to the platform providers. For example, the Facebook study (Cheng et al, 2014) was co-authored by two Facebook research scientists. Major conferences where this kind of research is presented are widely frequented by researchers with industry ties.
This raises important questions for the future. It is no coincidence that all three studies use very large amounts of data. Hong et al analyzed 62 million tweets in 100 different languages, Kwak et al mined 42 million user profiles and 1,48 billion social relations in their study, which now seems comparably dated given how strongly Twitter has grown since. And Cheng et al used 150,000 photos on Facebook that were shared over nine million times. This is not just an issue of using a lot of data, but about the granularity that combining different sources of information brings to the analysis, allowing increasingly precise predictions. The success of a cascade cannot be tied to any single factor, especially not causally. But the ability to predict the future shape of a cascade in real time already exists in basic terms.
This development is a major challenge for academic research, not just in relation to the privacy of users. Privileged access to research data is increasingly a competitive advantage, as the recently launched Twitter data grants illustrate, through which six international teams of academics have received the opportunity to work with data directly from the platform provider. The shift of emphasis from an explanatory to a predictive paradigm is a second challenge – in web science, basically everything is a multivariate problem that resists simple monocausal explanations. The social sciences are challenged to respond to the complex legal, ethical, and epistemological questions that this raises – if not in real time than at least in a more timely fashion than has been the case to date.
Sources
- Cheng, J., Adamic, L. A., Dow, P. A., Kleinberg, J., & Leskovec, J. (2014). Can cascades be predicted? In W. Lee, H.-C. Rim, & D. Schwabe (Eds.), Proceedings of the 23rd International World Wide Web Conference (WWW ’14) (pp. 1–11). Seoul, Republic of Korea: ACM Press. doi:10.1145/2566486.2567997
- Hong, L., Convertino, G., & Chi, E. H. (2011). Language matters in Twitter: A large scale study characterizing the top languages in Twitter characterizing differences across languages including URLs and hashtags. In Proceedings of the Fifth International AAAI Conference on Weblogs and Social Media (ICWSM ’11) (pp. 518–521). Menlo Park, CA: The AAAI Press.
- Kwak, H., Lee, C., Park, H., & Moon, S. (2010). What is Twitter, a social network or a news media? Categories and subject descriptors. In J. Freire & S. Chakrabarti (Eds.), Proceedings of the 19th International Conference on the World Wide Web (WWW ’10) (pp. 591–600). Raleigh, NC: ACM Press.
The author of this post is Cornelius Puschmann, associated researcher of the Alexander von Humboldt Institute for Internet and Society. The post does not necessarily represent the view of the Institute itself. For more information about the topics of these articles and associated research projects, please contact presse@hiig.de.
This post represents the view of the author and does not necessarily represent the view of the institute itself. For more information about the topics of these articles and associated research projects, please contact info@hiig.de.
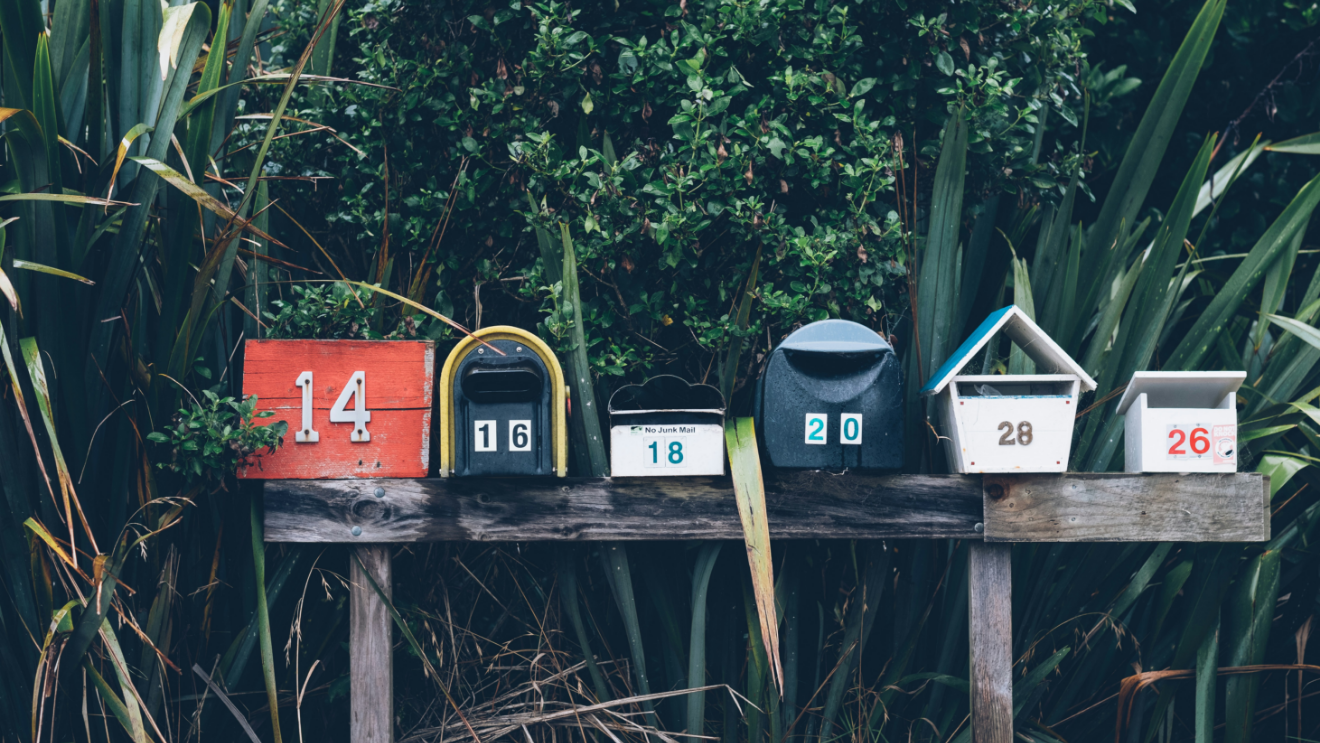
You will receive our latest blog articles once a month in a newsletter.
Research issues in focus
AI at the microphone: The voice of the future?
From synthesising voices and generating entire episodes, AI is transforming digital audio. Explore the opportunities and challenges of AI at the microphone.
Do Community Notes have a party preference?
This article explores whether Community Notes effectively combat disinformation or mirror political biases, analysing distribution and rating patterns.
How People Analytics can affect the perception of fairness in the workplace
People Analytics in the workplace can improve decisions but may also heighten feelings of unfairness, impacting employee trust and workplace relationships.