Making sense of our connected world
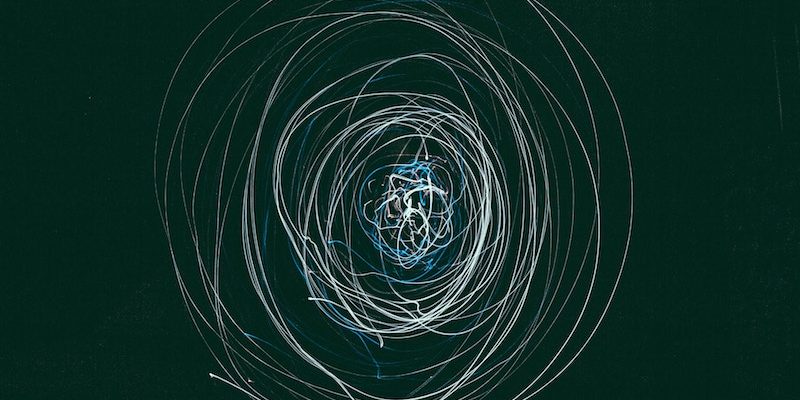
Understanding Artificial Intelligence
Artificial intelligence is a controversial and much-discussed topic of our digital future. Aljoscha Burchardt sees a huge gap between the machines of today, which are able to act intelligently, and the quest for actually being intelligent in a truly human sense. Together with Julia Ebert, he discussed the perspectives and challenges in the field.
Julia Ebert: The definition of artificial intelligence (AI) is controversial to this day. Intelligence itself is not easy to define – human intelligence and understanding the human brain still leaves many questions unresolved. What is artificial intelligence from your point of view? What does machine learning have to do with human intelligence?
Aljoscha Burchardt: Artificial intelligence has to do with a number of factors such as language understanding, sensing and being aware of your surroundings, planning, acting and of course learning. Only if several of these factors come together would I be tempted to say that a system or machine has some kind of intelligence. Machine learning is a technique that is used to model intelligent behaviour.
What was your motivation for going into the field of language technology and AI? Which main research questions have accompanied you?
When I learned grammar and foreign languages in school I was always irritated that everything teachers taught about language was soft in a way. It seemed that apart from grammatical exceptions there were no strict rules. My interest in a more mathematical approach to language brought me to the research field of computation and linguistics. Language is such a fascinating interface between humans and human knowledge – and even today, we still don’t understand how it really works. Most of the time, human learning processes are so effortless, especially when you look at small children. Actually we have no idea how we can teach machines with the same ease, efficiency and effectiveness. A child that has seen three dogs has understood the concept. We are trying to get our feet a little bit on the ground concerning machine learning but that is just the tip of the iceberg.
Looking at the current state of AI and machine learning, machines are basically copying human behaviour, e.g. in the case of autonomous driving or smart translation systems. So in short, in what way are we dealing with intelligent programs which are not capable of understanding their actions? How far will the next generation of AI be able to understand, based on acquired knowledge?
It’s a huge step between acting intelligently like machines do today and being truly intelligent. For example, a statistical translation engine is not intelligent in a human sense: it doesn’t “know” why it should translate a specific word in some context in a certain way – it simply does it. The same goes for a machine that has learnt how to label objects in a picture, e.g. in image search on the web. Of course the machine can label an object but it can’t say what this flower smells like or how much the car costs, etc. Don’t get me wrong; the systems can really do amazing things on huge amounts of data in almost no time. But the gap between performing tasks like translating or labelling and having common sense like a four-year-old child is really big. That is why it is so important to have a human in the loop. Look at applications in the medical field, for example cancer screening: the dermatologist can derive a comprehensive diagnosis that takes into account what an algorithm has learnt from the data collected on thousands of screened birthmarks. But besides the result on some scale there is no further explanation by the machine. In the next generation of machine learning, machines will hopefully provide an explanation that will make it possible to retrace how the machine got to its conclusion by looking at various characteristics – in this case, colour, shape etc. – and what it has learned from the data. But still, this would be far away from providing any medical or biological explanation. The same in the field of autonomous driving: an autonomous car stops because there is an obstacle ahead – no matter if it’s a child or a plastic bag. It doesn’t know why it has to brake. Concerning the most advanced phase of machine learning with machines that really know – in a human way of knowing – what they are doing, I have not the faintest idea how we will ever get there.
Through the most recent developments of deep learning significant progress has been made in language technology, especially translation quality. How does deep learning work?
Deep learning is a statistical way of implementing machine learning. It is based on a mathematical structure inspired by the design of our human brain: neurons and links between neurons that are organised in layers. The machine works within an input-output scheme and learns which features and which connections are helpful to perform the task. There is no more manual work to be done and so the system calibrates itself. It might seem like a black box for many people but I think it is in a way comparable with many technologies: I don’t know how my smartphone works inside but I accept it because it does what I want.
Creativity is probably one of the human abilities most difficult to automate. To what extent will machines be able to exhibit truly creative behaviour in future? Also, against the background of controllability of machines, what form of creativity is possible and reasonable?
I am convinced that machines cannot be creative in the same way that artists can be creative. Creating some new metaphor, style, images – this all has to do a lot with knowledge about cultural habits, dos and don’ts, expectations, etc. It’s the same with humour, which doesn’t even work cross-culturally. If we deal with machines, we want to control machines. But machines learn from the data we produce, they read through our articles and Wikipedia entries. So, it has happened that a chatbot learned a national-socialist jargon – this actually occurred when it was being used by mean users. It’s difficult when dealing with machine learning to act in a normative way. The only thing one can do is to try to create and collect balanced and neutral data. That is the responsibility of data scientists. We humans have to be a good example to the machines and of course we need human control mechanisms. Concerning this matter, I talked to a person from a big insurance company lately. They modelled hundreds of features of people’s lives, like their living conditions, incomes or other insurance policies, as features to model the decision whether they could grant them an insurance policy or not. Then, an algorithm was trained on the previous human decisions and learned to take the decision quite well. Later on they checked on what grounds the algorithm made its decisions: the only one feature the machine used was the geolocation. So people from a poor neighbourhood tend to not to get the insurance but people from rich neighbourhoods had a high probability of getting the insurance. From the machine perspective, this was a useful basis for the decisions, because it is simple and works in most cases. This example shows that we need human control, especially in single, potentially problematic machine decisions. There we need to find a practicable mode of human-machine cooperation. And here again; it would be a great achievement if a machine would be able to explain why it came to a certain decision so that we could decide whether it was a good reason.
There are few topics as filled with hope and hysteria as AI and its impact on our daily lives. Where do you see that AI can deliver the benefits many have predicted? Where are the limits? To what extent can we trust AI?
People are not tempted to trust machines. Machines can seemingly take decisions that we don’t understand. We understand if a tire breaks and the car goes in the wrong direction, as it is a physical problem which can be traced back. But if an algorithm steers an autonomous car in the wrong direction because there was some light or leaves on the camera, it is hard to accept this because you can’t trace the decision back. There is less fault tolerance of machines than humans. I’ve seen this from human translators when they get machine translations: if there is a very stupid mistake in one sentence, the user tends to lose confidence in the machine right away – even if the next 100 sentences would have been translated perfectly. In the case of a human, this would probably be advisable: If someone makes such a stupid mistake, it is probably a good idea not to hire him as a translator. We need to find ways to establish trust with machines. But the question is from which point would one person trust a machine? Does it take one hour of driving, one day, one week or a month before I give the same trust to an autonomous car? With humans we are absolutely trained in assessing a human’s capability in milliseconds. The same with physical objects: certificates and tests tell us what we can expect. Algorithms are so new that we have not yet learned when we can trust them.
Aljoscha Burchardt is lab manager at the Language Technology Lab of the German Research Center for Artificial Intelligence (DFKI). With a background in semantic language technology, his interests include the evaluation of (machine) translation quality and the inclusion of language professionals in the machine translation R&D workflow.
Julia Ebert is affiliated at DFKI and member of encore’s editorial team. This interview has also been published in Volume 2017 of encore – our annual magazine on internet and society research.
This post represents the view of the author and does not necessarily represent the view of the institute itself. For more information about the topics of these articles and associated research projects, please contact info@hiig.de.
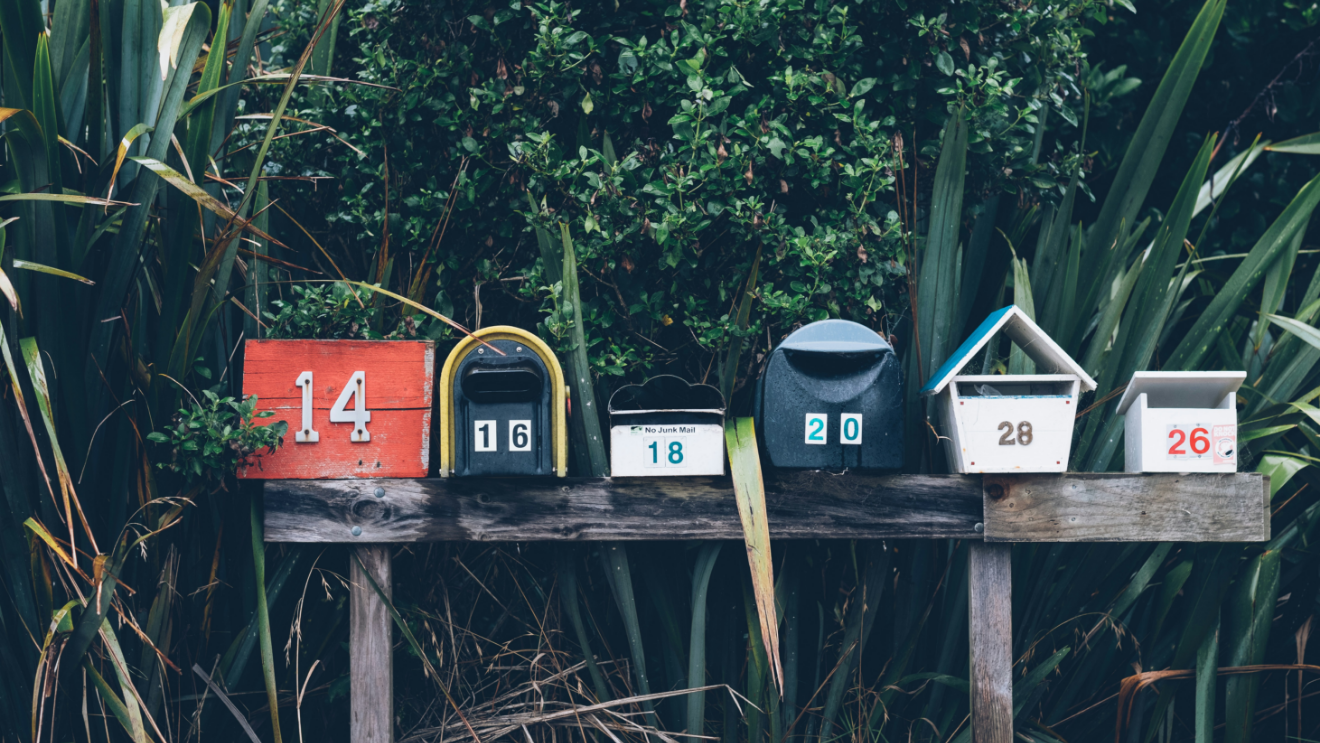
You will receive our latest blog articles once a month in a newsletter.
Research issues in focus
Why access rights to platform data for researchers restrict, not promote, academic freedom
New German and EU digital laws grant researchers access rights to platform data, but narrow definitions of research risk undermining academic freedom.
Empowering workers with data
As workplaces become data-driven, can workers use people analytics to advocate for their rights? This article explores how data empowers workers and unions.
Two years after the takeover: Four key policy changes of X under Musk
This article outlines four key policy changes of X since Musk’s 2022 takeover, highlighting how the platform's approach to content moderation has evolved.