Making sense of our connected world
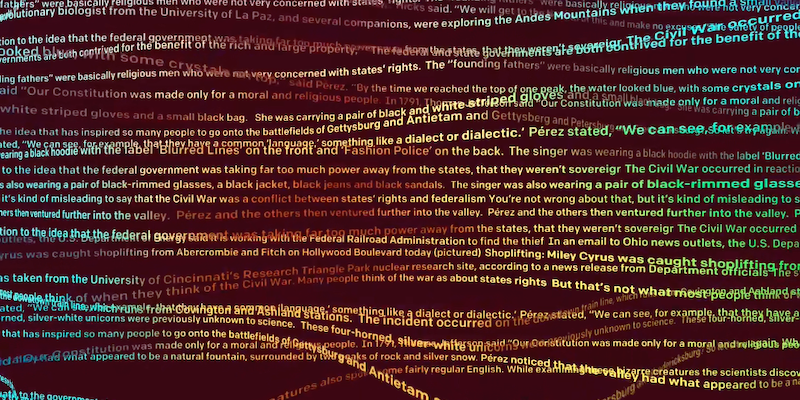
When will the first AI top the New York Times bestseller list?
An investigation of language-generating algorithms and their impact on society. Language generators based on artificial intelligence are producing increasingly convincing texts. This led us to wonder: Could an AI guest-author write an article in our annual encore magazine? The AI-written text you will see below is the result of our experiment.
This is what we did: the first paragraph is a human-written prompt. It is based on the introduction to HIIG researcher Alexander Pirang’s blog post “Is the COVID-19 pandemic social media platforms’ chance at redemption?” published on the Digital Society Blog in April 2020. The prompt was fed to InferKit, which offers an accessible version of the language model GPT-2. Released by OpenAI in 2019, this language model generates articles, poems or even code based on minimal prompts.
Does the result convince you? To keep things raw and provide a better idea of how the technology works, the AI-written piece below remains fully unedited. Finally, three HIIG researchers discuss the results and the potential impact of recently developed language-generating technologies.
The result
Human-written prompt: Is the COVID-19 pandemic social media platforms’ chance at redemption? Faced with an infodemic in the wake of COVID-19, social media platforms have ramped up their efforts to address misinformation. Facebook in particular seems to have implemented a surprisingly robust coronavirus response. These efforts could reinforce a public value-driven approach to content governance, but they could also be problems posing as solutions.
AI-written output: As social media platforms monitor users’ interactions, can they track and manage extremist content more effectively? This requires greater transparency, transparency that has so far been lacking. We are seeing much more transparency today, but most of it is being framed through terms that have been harnessed by proponents of transparency, making it difficult for third parties to understand.
Prior to COVID-19, Facebook (NASDAQ: FB) had faced concern about the quality and impact of their news feed. Users who wanted to stay informed were not being exposed to high quality, trustworthy content. Following COVID-19, there has been intense media scrutiny on Facebook, which may further influence its users to actively seek out reliable news sources. Whatever changes Facebook implements, how effective will they be? Based on the problems Facebook has had with extremism on its platform, can it really be trusted to make these changes?
In a similar vein, Twitter has arguably already faced unprecedented levels of scrutiny for its role in monitoring and policing the content on its platform. Twitter’s role in monitoring content, in contrast to Facebook’s, has focused more on fear-mongering and inflammatory commentary that has given rise to a series of debates over what constitutes a hate-filled tweet and who is ultimately responsible for the content posted on Twitter.
Facebook’s role in supporting the election of Donald Trump has created an environment of mistrust between the social media company and its users. While a majority of people who liked Donald Trump on Facebook have affirmed that their decision was based on his platform, which in turn led to the popularisation of the term “Trump Truther”, how effective can Facebook be in correcting the damage of its recent election support and how will it manage those who have made a poor choice?
It is important that Twitter continues to show leadership by removing the offensive tweets and deactivating the accounts that were created as a result of the election of Donald Trump. The removal of the offending tweets and support for the ban of Milo Yiannopoulos should signal that Twitter will resist attempts to influence users’ choices with hate speech and that it will do all it can to support free speech.
The context
HIIG researchers Shlomi Hod, Alexander Pirang and Frédéric Dubois were asked to provide perspectives from their fields of expertise on the article written by the AI guest author. Shlomi is a visiting researcher at HIIG’s AI & Society Lab and computer science PhD candidate at Boston University. Alexander was a researcher at HIIG, currently writing his doctoral thesis on the implications of platform governance for users’ right to freedom of expression. Frédéric is managing editor of HIIG’s Internet Policy Review and PhD candidate at the Film University Babelsberg. The interview was conducted by Sonja Köhne.
The interview
Sonja: From a technical perspective, how did the AI text generator arrive at this result?
Shlomi: The GPT-2 is a language model designed to predict the next word given a context, namely all the previous words in the text so far. It was trained using a large dataset of text from over 8 million web pages in English that Reddit users shared. The model is based on a recent neural network architecture from 2017, called the Transformer, which had a huge impact on the field of NLP (natural language processing), with great advances from 2019 on. The Transformer is built out of a series of self-attention mechanisms that allow it to process the input text by focusing or paying attention to different words in the sentence simultaneously.
Alexander, you wrote the blog post that we used as a prompt. Were you surprised reading the AI-generated text?
Alexander: At first glance, the text seemed surprisingly coherent and even eloquently written. Many of the word choices, like “monitoring and policing content”, are used by researchers and journalists all the time. The frequent use of open-ended questions also struck me as an effective way to engage with the topic while avoiding stronger statements. Yet, it does not take long to notice the wrinkles. Some of the arguments are little more than words piled on top of other words: who are the proponents of transparency mentioned and why does their harnessing of transparency-related terms frame the issue so as to impede third parties’ understanding? Unfortunately, no clues are given. In a way, the piece resembles a collage of general discussion points about the challenges of harmful content and the role of social media in the US presidential election.
To what extent did you approach the topic differently in the original blog post?
Alexander: In the piece I wrote back in April 2020, I cautioned that the measures rolled out by social media platforms in the wake of COVID-19 should not be seen as a panacea, as concerns remained about platforms’ opaque content governance processes and problematic gatekeeping functions. This specific perspective was lost in the AI-generated text, which only fleetingly mentioned COVID-19.
From an editor’s perspective, how does the style of writing read?
Frédéric: To me this text reads like a poorly written piece of unedited text… Or rather, as a bad text originally written in another language, which was then put through a first-generation online translating tool. The writing style might qualify as a hastily written and uninformed opinion article. Beyond style, though, the substance slaloms between misleading (e.g. sentences such as “Users who wanted to stay informed were not being exposed to high quality, trustworthy content” are stated in absolute terms, with no space for nuance) and quite accurate parts (e.g. the paragraph about Twitter), then again zapping to generalist phrases that leave out basic context (e.g. the story of users’ trust in Facebook and Twitter when it comes to political content is much broader and would need to refer to at least basics such as the Facebook–Cambridge Analytica scandal).
What promising projects are underway in the field of language generation? Is the technology likely to improve significantly in the coming years?
Shlomi: Before we rush to (carefully) imagine the future, the current progress is already impressive. GPT-3, the successor of GPT-2, which has a vastly greater number of parameters, was published in summer 2020. The model was not released to the public; you had to apply for access. Demonstration of its ability and application stormed the internet shortly after its announcement, and it achieved state-of-the-art results in multiple NLP tasks. In fact, it managed to perform well in tasks that it was not explicitly trained for, only through showing it a few examples in the input text. Suppose we feed GPT-3 with a few sentences in English and their German translation. Then finally we insert the English sentence we want to translate – there is a good chance that GPT-3 will succeed!
Where can this technology be applied in practice?
Shlomi: Back when GPT-2 was released, it was the largest Transformer-trained language model, consisting of 1.5 billion parameters (i.e. the values that the model needs to learn), and indeed, it exhibits impressive ability for a machine to generate relatively high-quality text, albeit far from what a professional writer would produce. GPT-2 and other recent language models are useful not only for generating text in an article form but also for other tasks involving human language, such as the classification of texts in categories, analysing a text’s sentiment and powering chatbot dialogue.
In September 2020, The Guardian asked GPT-3, OpenAI’s powerful new text generator, to write an essay from scratch. Editing the op-ed by GPT-3 was no different from editing a human op-ed, according to The Guardian. Lines were cut off and paragraphs were rearranged – in fact the process took less time than editing many human op-eds. So this tool is of course impressive, but it still has some weaknesses. What opportunities, but also dangers, can be associated with its popular usage and easy access, e.g. in journalism?
Frédéric: As machine learning algorithms get more sophisticated and dataset sources diversify exponentially, I expect machine learning articles to become if not dominant, well-represented in generalist news media. This is the natural next step and builds on a long tradition of journalism automation. Newswire services already homogenise news worldwide daily. What will be key moving forward is for schools to teach critical media reading, editors to be even more alert and for human-led quality journalism to grow and show resilience.
How can a text generator be sure that it is fed with credible sources? Can this tool distinguish truth from falsity – or detect harmful biases?
Shlomi: The GPT-2 language model is not designed to distinguish between credible and non-credible sources. Interestingly, researchers from Allen Institute for AI and the University of Washington developed a fake news text generator. They found out that the best way to identify whether a text was generated by humans or the model is by using a variant of the model itself! It suggests that building strong language models and machine-text detectors go hand-in-hand.
The evaluation of statistical language models and their neural networks with scientific standards remains a challenge. Even the developers cannot always understand why the AI generates what it does. This touches on a fundamental epistemological question: how do we actually learn to recognise meaning?
Shlomi: We should keep in mind that these models do not understand the world as we do. Their way of capturing language is through the complex relationship between words, not necessarily through understanding the words themselves and their relation to the real world.
This text was first published in our research magazine encore.
This post represents the view of the author and does not necessarily represent the view of the institute itself. For more information about the topics of these articles and associated research projects, please contact info@hiig.de.
Artificial intelligence and society
Sign up for HIIG's Monthly Digest
and receive our latest blog articles.
Debunking Science Myths: Preconceptions about science put to the test
What is really true about preconceptions about science? Four popular myths about a constantly quarrelling group of professionals explained simply.
No Smartphone = Cringe Weirdo
In this blog post, author Jascha Bareis shares his experiences since getting his first smartphone just this year.
European elections and digital policy: German party positions
To what extent are German parties addressing digital policy in the European elections? A glance at the election programmes reveals different priorities.